More on Entrepreneurship/Creators

Micah Daigle
2 years ago
Facebook is going away. Here are two explanations for why it hasn't been replaced yet.
And tips for anyone trying.
We see the same story every few years.
BREAKING NEWS: [Platform X] launched a social network. With Facebook's reputation down, the new startup bets millions will switch.
Despite the excitement surrounding each new platform (Diaspora, Ello, Path, MeWe, Minds, Vero, etc.), no major exodus occurred.
Snapchat and TikTok attracted teens with fresh experiences (ephemeral messaging and rapid-fire videos). These features aren't Facebook, even if Facebook replicated them.
Facebook's core is simple: you publish items (typically text/images) and your friends (generally people you know IRL) can discuss them.
It's cool. Sometimes I don't want to, but sh*t. I like it.
Because, well, I like many folks I've met. I enjoy keeping in touch with them and their banter.
I dislike Facebook's corporation. I've been cautiously optimistic whenever a Facebook-killer surfaced.
None succeeded.
Why? Two causes, I think:
People couldn't switch quickly enough, which is reason #1
Your buddies make a social network social.
Facebook started in self-contained communities (college campuses) then grew outward. But a new platform can't.
If we're expected to leave Facebook, we want to know that most of our friends will too.
Most Facebook-killers had bottlenecks. You have to waitlist or jump through hoops (e.g. setting up a server).
Same outcome. Upload. Chirp.
After a week or two of silence, individuals returned to Facebook.
Reason #2: The fundamental experience was different.
Even when many of our friends joined in the first few weeks, it wasn't the same.
There were missing features or a different UX.
Want to reply with a meme? No photos in comments yet. (Trying!)
Want to tag a friend? Nope, sorry. 2019!
Want your friends to see your post? You must post to all your friends' servers. Good luck!
It's difficult to introduce a platform with 100% of the same features as one that's been there for 20 years, yet customers want a core experience.
If you can't, they'll depart.
The causes that led to the causes
Having worked on software teams for 14+ years, I'm not surprised by these challenges. They are a natural development of a few tech sector meta-problems:
Lean startup methodology
Silicon Valley worships lean startup. It's a way of developing software that involves testing a stripped-down version with a limited number of people before selecting what to build.
Billion people use Facebook's functions. They aren't tested. It must work right away*
*This may seem weird to software people, but it's how non-software works! You can't sell a car without wheels.
2. Creativity
Startup entrepreneurs build new things, not copies. I understand. Reinventing the wheel is boring.
We know what works. Different experiences raise adoption friction. Once millions have transferred, more features (and a friendlier UX) can be implemented.
3. Cost scaling
True. Building a product that can sustain hundreds of millions of users in weeks is expensive and complex.
Your lifeboats must have the same capacity as the ship you're evacuating. It's required.
4. Pure ideologies
People who work on Facebook-alternatives are (understandably) critical of Facebook.
They build an open-source, fully-distributed, data-portable, interface-customizable, offline-capable, censorship-proof platform.
Prioritizing these aims can prevent replicating the straightforward experience users expect. Github, not Facebook, is for techies only.
What about the business plan, though?
Facebook-killer attempts have followed three models.
Utilize VC funding to increase your user base, then monetize them later. (If you do this, you won't kill Facebook; instead, Facebook will become you.)
Users must pay to utilize it. (This causes a huge bottleneck and slows the required quick expansion, preventing it from seeming like a true social network.)
Make it a volunteer-run, open-source endeavor that is free. (This typically denotes that something is cumbersome, difficult to operate, and is only for techies.)
Wikipedia is a fourth way.
Wikipedia is one of the most popular websites and a charity. No ads. Donations support them.
A Facebook-killer managed by a good team may gather millions (from affluent contributors and the crowd) for their initial phase of development. Then it might sustain on regular donations, ethical transactions (e.g. fees on commerce, business sites, etc.), and government grants/subsidies (since it would essentially be a public utility).
When you're not aiming to make investors rich, it's remarkable how little money you need.
If you want to build a Facebook competitor, follow these tips:
Drop the lean startup philosophy. Wait until you have a finished product before launching. Build it, thoroughly test it for bugs, and then release it.
Delay innovating. Wait till millions of people have switched before introducing your great new features. Make it nearly identical for now.
Spend money climbing. Make sure that guests can arrive as soon as they are invited. Never keep them waiting. Make things easy for them.
Make it accessible to all. Even if doing so renders it less philosophically pure, it shouldn't require technical expertise to utilize.
Constitute a nonprofit. Additionally, develop community ownership structures. Profit maximization is not the only strategy for preserving valued assets.
Last thoughts
Nobody has killed Facebook, but Facebook is killing itself.
The startup is burying the newsfeed to become a TikTok clone. Meta itself seems to be ditching the platform for the metaverse.
I wish I was happy, but I'm not. I miss (understandably) removed friends' postings and remarks. It could be a ghost town in a few years. My dance moves aren't TikTok-worthy.
Who will lead? It's time to develop a social network for the people.
Greetings if you're working on it. I'm not a company founder, but I like to help hard-working folks.

ʟ ᴜ ᴄ ʏ
2 years ago
The Untapped Gold Mine of Inspiration and Startup Ideas
I joined the 1000 Digital Startups Movement (Gerakan 1000 Startup Digital) in 2017 and learned a lot about the startup sector. My previous essay outlined what a startup is and what must be prepared. Here I'll offer raw ideas for better products.
Intro
A good startup solves a problem. These can include environmental, economic, energy, transportation, logistics, maritime, forestry, livestock, education, tourism, legal, arts and culture, communication, and information challenges. Everything I wrote is simply a basic idea (as inspiration) and requires more mapping and validation. Learn how to construct a startup to maximize launch success.
Adrian Gunadi (Investree Co-Founder) taught me that a Founder or Co-Founder must be willing to be CEO (Chief Everything Officer). Everything is independent, including drafting a proposal, managing finances, and scheduling appointments. The best individuals will come to you if you're the best. It's easier than consulting Andy Zain (Kejora Capital Founder).
Description
To help better understanding from your idea, try to answer this following questions:
- Describe your idea/application
Maximum 1000 characters.
- Background
Explain the reasons that prompted you to realize the idea/application.
- Objective
Explain the expected goals of the creation of the idea/application.
- Solution
A solution that tells your idea can be the right solution for the problem at hand.
- Uniqueness
What makes your idea/app unique?
- Market share
Who are the people who need and are looking for your idea?
- Marketing Ways and Business Models
What is the best way to sell your idea and what is the business model?
Not everything here is a startup idea. It's meant to inspire creativity and new perspectives.
Ideas
#Application
1. Medical students can operate on patients or not. Applications that train prospective doctors to distinguish body organs and their placement are useful. In the advanced stage, the app can be built with numerous approaches so future doctors can practice operating on patients based on their ailments. If they made a mistake, they'd start over. Future doctors will be more assured and make fewer mistakes this way.
2. VR (virtual reality) technology lets people see 3D space from afar. Later, similar technology was utilized to digitally sell properties, so buyers could see the inside and room contents. Every gadget has flaws. It's like a gold mine for robbers. VR can let prospective students see a campus's facilities. This facility can also help hotels promote their products.
3. How can retail entrepreneurs maximize sales? Most popular goods' sales data. By using product and brand/type sales figures, entrepreneurs can avoid overstocking. Walmart computerized their procedures to track products from the manufacturer to the store. As Retail Link products sell out, suppliers can immediately step in.
4. Failing to marry is something to be avoided. But if it had to happen, the loss would be like the proverb “rub salt into the wound”. On the I do Now I dont website, Americans who don't marry can resell their jewelry to other brides-to-be. If some want to cancel the wedding and receive their down money and dress back, others want a wedding with particular criteria, such as a quick date and the expected building. Create a DP takeover marketplace for both sides.
#Games
1. Like in the movie, players must exit the maze they enter within 3 minutes or the shape will change, requiring them to change their strategy. The maze's transformation time will shorten after a few stages.
2. Treasure hunts involve following clues to uncover hidden goods. Here, numerous sponsors are combined in one boat, and participants can choose a game based on the prizes. Let's say X-mart is a sponsor and provides riddles or puzzles to uncover the prize in their store. After gathering enough points, the player can trade them for a gift utilizing GPS and AR (augmented reality). Players can collaborate to increase their chances of success.
3. Where's Wally? Where’s Wally displays a thick image with several things and various Wally-like characters. We must find the actual Wally, his companions, and the desired object. Make a game with a map where players must find objects for the next level. The player must find 5 artifacts randomly placed in an Egyptian-style mansion, for example. In the room, there are standard tickets, pass tickets, and gold tickets that can be removed for safekeeping, as well as a wall-mounted carpet that can be stored but not searched and turns out to be a flying rug that can be used to cross/jump to a different place. Regular tickets are spread out since they can buy life or stuff. At a higher level, a black ticket can lower your ordinary ticket. Objects can explode, scattering previously acquired stuff. If a player runs out of time, they can exchange a ticket for more.
#TVprogram
1. At the airport there are various visitors who come with different purposes. Asking tourists to live for 1 or 2 days in the city will be intriguing to witness.
2. Many professions exist. Carpenters, cooks, and lawyers must have known about job desks. Does HRD (Human Resource Development) only recruit new employees? Many don't know how to become a CEO, CMO, COO, CFO, or CTO. Showing young people what a Program Officer in an NGO does can help them choose a career.
#StampsCreations
Philatelists know that only the government can issue stamps. I hope stamps are creative so they have more worth.
1. Thermochromic pigments (leuco dyes) are well-known for their distinctive properties. By putting pigments to black and white batik stamps, for example, the black color will be translucent and display the basic color when touched (at a hot temperature).
2. In 2012, Liechtenstein Post published a laser-art Chinese zodiac stamp. Belgium (Bruges Market Square 2012), Taiwan (Swallow Tail Butterfly 2009), etc. Why not make a stencil of the president or king/queen?
3. Each country needs its unique identity, like Taiwan's silk and bamboo stamps. Create from your country's history. Using traditional paper like washi (Japan), hanji (Korea), and daluang/saeh (Indonesia) can introduce a country's culture.
4. Garbage has long been a problem. Bagasse, banana fronds, or corn husks can be used as stamp material.
5. Austria Post published a stamp containing meteor dust in 2006. 2004 meteorite found in Morocco produced the dust. Gibraltar's Rock of Gilbraltar appeared on stamps in 2002. What's so great about your country? East Java is muddy (Lapindo mud). Lapindo mud stamps will be popular. Red sand at Pink Beach, East Nusa Tenggara, could replace the mud.
#PostcardCreations
1. Map postcards are popular because they make searching easier. Combining laser-cut road map patterns with perforated 200-gram paper glued on 400-gram paper as a writing medium. Vision-impaired people can use laser-cut maps.
2. Regional art can be promoted by tucking traditional textiles into postcards.
3. A thin canvas or plain paper on the card's front allows the giver to be creative.
4. What is local crop residue? Cork lids, maize husks, and rice husks can be recycled into postcard materials.
5. Have you seen a dried-flower bookmark? Cover the postcard with mica and add dried flowers. If you're worried about losing the flowers, you can glue them or make a postcard envelope.
6. Wood may be ubiquitous; try a 0.2-mm copper plate engraved with an image and connected to a postcard as a writing medium.
7. Utilized paper pulp can be used to hold eggs, smartphones, and food. Form a smooth paper pulp on the plate with the desired image, the Golden Gate bridge, and paste it on your card.
8. Postcards can promote perfume. When customers rub their hands on the card with the perfume image, they'll smell the aroma.
#Tour #Travel
Tourism activities can be tailored to tourists' interests or needs. Each tourist benefits from tourism's distinct aim.
Let's define tourism's objective and purpose.
Holiday Tour is a tour that its participants plan and do in order to relax, have fun, and amuse themselves.
A familiarization tour is a journey designed to help travelers learn more about (survey) locales connected to their line of work.
An educational tour is one that aims to give visitors knowledge of the field of work they are visiting or an overview of it.
A scientific field is investigated and knowledge gained as the major goal of a scientific tour.
A pilgrimage tour is one designed to engage in acts of worship.
A special mission tour is one that has a specific goal, such a commerce mission or an artistic endeavor.
A hunting tour is a destination for tourists that plans organized animal hunting that is only allowed by local authorities for entertainment purposes.
Every part of life has tourism potential. Activities include:
1. Those who desire to volunteer can benefit from the humanitarian theme and collaboration with NGOs. This activity's profit isn't huge but consider the environmental impact.
2. Want to escape the city? Meditation travel can help. Beautiful spots around the globe can help people forget their concerns. A certified yoga/meditation teacher can help travelers release bad energy.
3. Any prison visitors? Some prisons, like those for minors under 17, are open to visitors. This type of tourism helps mental convicts reach a brighter future.
4. Who has taken a factory tour/study tour? Outside-of-school study tour (for ordinary people who have finished their studies). Not everyone in school could tour industries, workplaces, or embassies to learn and be inspired. Shoyeido (an incense maker) and Royce (a chocolate maker) offer factory tours in Japan.
5. Develop educational tourism like astronomy and archaeology. Until now, only a few astronomy enthusiasts have promoted astronomy tourism. In Indonesia, archaeology activities focus on site preservation, and to participate, office staff must undertake a series of training (not everyone can take a sabbatical from their routine). Archaeological tourist activities are limited, whether held by history and culture enthusiasts or in regional tours.
6. Have you ever longed to observe a film being made or your favorite musician rehearsing? Such tours can motivate young people to pursue entertainment careers.
7. Pamper your pets to reduce stress. Many pet owners don't have time for walks or treats. These premium services target the wealthy.
8. A quirky idea to provide tours for imaginary couples or things. Some people marry inanimate objects or animals and seek to make their lover happy; others cherish their ashes after death.
#MISCideas
1. Fashion is a lifestyle, thus people often seek fresh materials. Chicken claws, geckos, snake skin casings, mice, bats, and fish skins are also used. Needs some improvement, definitely.
2. As fuel supplies become scarcer, people hunt for other energy sources. Sound is an underutilized renewable energy. The Batechsant technology converts environmental noise into electrical energy, according to study (Battery Technology Of Sound Power Plant). South Korean researchers use Sound-Driven Piezoelectric Nanowire based on Nanogenerators to recharge cell phone batteries. The Batechsant system uses existing noise levels to provide electricity for street lamp lights, aviation, and ships. Using waterfall sound can also energize hard-to-reach locations.
3. A New York Times reporter said IQ doesn't ensure success. Our school system prioritizes IQ above EQ (Emotional Quotient). EQ is a sort of human intelligence that allows a person to perceive and analyze the dynamics of his emotions when interacting with others (and with himself). EQ is suspected of being a bigger source of success than IQ. EQ training can gain greater attention to help people succeed. Prioritize role models from school stakeholders, teachers, and parents to improve children' EQ.
4. Teaching focuses more on theory than practice, so students are less eager to explore and easily forget if they don't pay attention. Has an engineer ever made bricks from arid red soil? Morocco's non-college-educated builders can create weatherproof bricks from red soil without equipment. Can mechanical engineering grads create a water pump to solve water shortages in remote areas? Art graduates can innovate beyond only painting. Artists may create kinetic sculpture by experimenting so much. Young people should understand these sciences so they can be more creative with their potential. These might be extracurricular activities in high school and university.
5. People have been trying to recycle agricultural waste for a long time. Mycelium helps replace light, easily crushed tiles and bricks (a collection of hyphae like in the manufacture of tempe). Waste must contain lignocellulose. In this vein, anti-mainstream painting canvases can be made. The goal is to create the canvas uneven like an amoeba outline, not square or spherical. The resulting canvas is lightweight and needs no frame. Then what? Open source your idea like Precious Plastic to establish a community. By propagating this notion, many knowledgeable people will help improve your product's quality and impact.
6. As technology and humans adapt, fraud increases. Making phony doctor's letters to fool superiors, fake credentials to get hired, fraudulent land certificates to make money, and fake news (hoax). The existence of a Wikimedia can aid the community by comparing bogus and original information.
7. Do you often hit a problem-solving impasse? Since the Doraemon bag hasn't been made, construct an Idea Bank. Everyone can contribute to solving problems here. How do you recruit volunteers? Obviously, a reward is needed. Contributors can become moderators or gain complimentary tickets to TIA (Tech in Asia) conferences. Idea Bank-related concepts: the rise of startups without a solid foundation generates an age as old as corn that does not continue. Those with startup ideas should describe them here so they can be validated by other users. Other users can contribute input if a comparable notion is produced to improve the product or integrate it. Similar-minded users can become Co-Founders.
8. Why not invest in fruit/vegetables, inspired by digital farming? The landowner obtains free fruit without spending much money on maintenance. Investors can get fruits/vegetables in larger quantities, fresher, and cheaper during harvest. Fruits and vegetables are often harmed if delivered too slowly. Rich investors with limited land can invest in teak, agarwood, and other trees. When harvesting, investors might choose raw results or direct wood sales earnings. Teak takes at least 7 years to harvest, therefore long-term wood investments carry the risk of crop failure.
9. Teenagers in distant locations can't count, read, or write. Many factors hinder locals' success. Life's demands force them to work instead of study. Creating a learning playground may attract young people to learning. Make a skatepark at school. Skateboarders must learn in school. Donations buy skateboards.
10. Globally, online taxi-bike is known. By hiring a motorcycle/car online, people no longer bother traveling without a vehicle. What if you wish to cross the island or visit remote areas? Is online boat or helicopter rental possible like online taxi-bike? Such a renting process has been done independently thus far and cannot be done quickly.
11. What do startups need now? A startup or investor consultant. How many startups fail to become Unicorns? Many founders don't know how to manage investor money, therefore they waste it on promotions and other things. Many investors only know how to invest and can't guide a struggling firm.
“In times of crisis, the wise build bridges, while the foolish build barriers.” — T’Challa [Black Panther]
Don't chase cash. Money is a byproduct. Profit-seeking is stressful. Market requirements are opportunities. If you have something to say, please comment.
This is only informational. Before implementing ideas, do further study.

Sammy Abdullah
2 years ago
R&D, S&M, and G&A expense ratios for SaaS
SaaS spending is 40/40/20. 40% of operating expenses should be R&D, 40% sales and marketing, and 20% G&A. We wanted to see the statistics behind the rules of thumb. Since October 2017, 73 SaaS startups have gone public. Perhaps the rule of thumb should be 30/50/20. The data is below.
30/50/20. R&D accounts for 26% of opex, sales and marketing 48%, and G&A 22%. We think R&D/S&M/G&A should be 30/50/20.
There are outliers. There are exceptions to rules of thumb. Dropbox spent 45% on R&D whereas Zoom spent 13%. Zoom spent 73% on S&M, Dropbox 37%, and Bill.com 28%. Snowflake spent 130% of revenue on S&M, while their EBITDA margin is -192%.
G&A shouldn't stand out. Minimize G&A spending. Priorities should be product development and sales. Cloudflare, Sendgrid, Snowflake, and Palantir spend 36%, 34%, 37%, and 43% on G&A.
Another myth is that COGS is 20% of revenue. Median and averages are 29%.
Where is the profitability? Data-driven operating income calculations were simplified (Revenue COGS R&D S&M G&A). 20 of 73 IPO businesses reported operational income. Median and average operating income margins are -21% and -27%.
As long as you're growing fast, have outstanding retention, and marquee clients, you can burn cash since recurring income that doesn't churn is a valuable annuity.
The data was compelling overall. 30/50/20 is the new 40/40/20 for more established SaaS enterprises, unprofitability is alright as long as your business is expanding, and COGS can be somewhat more than 20% of revenue.
You might also like

middlemarch.eth
3 years ago
ERC721R: A new ERC721 contract for random minting so people don’t snipe all the rares!
That is, how to snipe all the rares without using ERC721R!
Introduction: Blessed and Lucky
Mphers was the first mfers derivative, and as a Phunks derivative, I wanted one.
I wanted an alien. And there are only 8 in the 6,969 collection. I got one!
In case it wasn't clear from the tweet, I meant that I was lucky to have figured out how to 100% guarantee I'd get an alien without any extra luck.
Read on to find out how I did it, how you can too, and how developers can avoid it!
How to make rare NFTs without luck.
# How to mint rare NFTs without needing luck
The key to minting a rare NFT is knowing the token's id ahead of time.
For example, once I knew my alien was #4002, I simply refreshed the mint page until #3992 was minted, and then mint 10 mphers.
How did I know #4002 was extraterrestrial? Let's go back.
First, go to the mpher contract's Etherscan page and look up the tokenURI of a previously issued token, token #1:
As you can see, mphers creates metadata URIs by combining the token id and an IPFS hash.
This method gives you the collection's provenance in every URI, and while that URI can be changed, it affects everyone and is public.
Consider a token URI without a provenance hash, like https://mphers.art/api?tokenId=1.
As a collector, you couldn't be sure the devs weren't changing #1's metadata at will.
The API allows you to specify “if #4002 has not been minted, do not show any information about it”, whereas IPFS does not allow this.
It's possible to look up the metadata of any token, whether or not it's been minted.
Simply replace the trailing “1” with your desired id.
Mpher #4002
These files contain all the information about the mpher with the specified id. For my alien, we simply search all metadata files for the string “alien mpher.”
Take a look at the 6,969 meta-data files I'm using OpenSea's IPFS gateway, but you could use ipfs.io or something else.
Use curl to download ten files at once. Downloading thousands of files quickly can lead to duplicates or errors. But with a little tweaking, you should be able to get everything (and dupes are fine for our purposes).
Now that you have everything in one place, grep for aliens:
The numbers are the file names that contain “alien mpher” and thus the aliens' ids.
The entire process takes under ten minutes. This technique works on many NFTs currently minting.
In practice, manually minting at the right time to get the alien is difficult, especially when tokens mint quickly. Then write a bot to poll totalSupply() every second and submit the mint transaction at the exact right time.
You could even look for the token you need in the mempool before it is minted, and get your mint into the same block!
However, in my experience, the “big” approach wins 95% of the time—but not 100%.
“Am I being set up all along?”
Is a question you might ask yourself if you're new to this.
It's disheartening to think you had no chance of minting anything that someone else wanted.
But, did you have no opportunity? You had an equal chance as everyone else!
Take me, for instance: I figured this out using open-source tools and free public information. Anyone can do this, and not understanding how a contract works before minting will lead to much worse issues.
The mpher mint was fair.
While a fair game, “snipe the alien” may not have been everyone's cup of tea.
People may have had more fun playing the “mint lottery” where tokens were distributed at random and no one could gain an advantage over someone simply clicking the “mint” button.
How might we proceed?
Minting For Fashion Hats Punks, I wanted to create a random minting experience without sacrificing fairness. In my opinion, a predictable mint beats an unfair one. Above all, participants must be equal.
Sadly, the most common method of creating a random experience—the post-mint “reveal”—is deeply unfair. It works as follows:
- During the mint, token metadata is unavailable. Instead, tokenURI() returns a blank JSON file for each id.
- An IPFS hash is updated once all tokens are minted.
- You can't tell how the contract owner chose which token ids got which metadata, so it appears random.
Because they alone decide who gets what, the person setting the metadata clearly has a huge unfair advantage over the people minting. Unlike the mpher mint, you have no chance of winning here.
But what if it's a well-known, trusted, doxxed dev team? Are reveals okay here?
No! No one should be trusted with such power. Even if someone isn't consciously trying to cheat, they have unconscious biases. They might also make a mistake and not realize it until it's too late, for example.
You should also not trust yourself. Imagine doing a reveal, thinking you did it correctly (nothing is 100%! ), and getting the rarest NFT. Isn't that a tad odd Do you think you deserve it? An NFT developer like myself would hate to be in this situation.
Reveals are bad*
UNLESS they are done without trust, meaning everyone can verify their fairness without relying on the developers (which you should never do).
An on-chain reveal powered by randomness that is verifiably outside of anyone's control is the most common way to achieve a trustless reveal (e.g., through Chainlink).
Tubby Cats did an excellent job on this reveal, and I highly recommend their contract and launch reflections. Their reveal was also cool because it was progressive—you didn't have to wait until the end of the mint to find out.
In his post-launch reflections, @DefiLlama stated that he made the contract as trustless as possible, removing as much trust as possible from the team.
In my opinion, everyone should know the rules of the game and trust that they will not be changed mid-stream, while trust minimization is critical because smart contracts were designed to reduce trust (and it makes it impossible to hack even if the team is compromised). This was a huge mistake because it limited our flexibility and our ability to correct mistakes.
And @DefiLlama is a superstar developer. Imagine how much stress maximizing trustlessness will cause you!
That leaves me with a bad solution that works in 99 percent of cases and is much easier to implement: random token assignments.
Introducing ERC721R: A fully compliant IERC721 implementation that picks token ids at random.
ERC721R implements the opposite of a reveal: we mint token ids randomly and assign metadata deterministically.
This allows us to reveal all metadata prior to minting while reducing snipe chances.
Then import the contract and use this code:
What is ERC721R and how does it work
First, a disclaimer: ERC721R isn't truly random. In this sense, it creates the same “game” as the mpher situation, where minters compete to exploit the mint. However, ERC721R is a much more difficult game.
To game ERC721R, you need to be able to predict a hash value using these inputs:
This is impossible for a normal person because it requires knowledge of the block timestamp of your mint, which you do not have.
To do this, a miner must set the timestamp to a value in the future, and whatever they do is dependent on the previous block's hash, which expires in about ten seconds when the next block is mined.
This pseudo-randomness is “good enough,” but if big money is involved, it will be gamed. Of course, the system it replaces—predictable minting—can be manipulated.
The token id is chosen in a clever implementation of the Fisher–Yates shuffle algorithm that I copied from CryptoPhunksV2.
Consider first the naive solution: (a 10,000 item collection is assumed):
- Make an array with 0–9999.
- To create a token, pick a random item from the array and use that as the token's id.
- Remove that value from the array and shorten it by one so that every index corresponds to an available token id.
This works, but it uses too much gas because changing an array's length and storing a large array of non-zero values is expensive.
How do we avoid them both? What if we started with a cheap 10,000-zero array? Let's assign an id to each index in that array.
Assume we pick index #6500 at random—#6500 is our token id, and we replace the 0 with a 1.
But what if we chose #6500 again? A 1 would indicate #6500 was taken, but then what? We can't just "roll again" because gas will be unpredictable and high, especially later mints.
This allows us to pick a token id 100% of the time without having to keep a separate list. Here's how it works:
- Make a 10,000 0 array.
- Create a 10,000 uint numAvailableTokens.
- Pick a number between 0 and numAvailableTokens. -1
- Think of #6500—look at index #6500. If it's 0, the next token id is #6500. If not, the value at index #6500 is your next token id (weird!)
- Examine the array's last value, numAvailableTokens — 1. If it's 0, move the value at #6500 to the end of the array (#9999 if it's the first token). If the array's last value is not zero, update index #6500 to store it.
- numAvailableTokens is decreased by 1.
- Repeat 3–6 for the next token id.
So there you go! The array stays the same size, but we can choose an available id reliably. The Solidity code is as follows:
Unfortunately, this algorithm uses more gas than the leading sequential mint solution, ERC721A.
This is most noticeable when minting multiple tokens in one transaction—a 10 token mint on ERC721R costs 5x more than on ERC721A. That said, ERC721A has been optimized much further than ERC721R so there is probably room for improvement.
Conclusion
Listed below are your options:
- ERC721A: Minters pay lower gas but must spend time and energy devising and executing a competitive minting strategy or be comfortable with worse minting results.
- ERC721R: Higher gas, but the easy minting strategy of just clicking the button is optimal in all but the most extreme cases. If miners game ERC721R it’s the worst of both worlds: higher gas and a ton of work to compete.
- ERC721A + standard reveal: Low gas, but not verifiably fair. Please do not do this!
- ERC721A + trustless reveal: The best solution if done correctly, highly-challenging for dev, potential for difficult-to-correct errors.
Did I miss something? Comment or tweet me @dumbnamenumbers.
Check out the code on GitHub to learn more! Pull requests are welcome—I'm sure I've missed many gas-saving opportunities.
Thanks!
Read the original post here

Matthew Cluff
2 years ago
GTO Poker 101
"GTO" (Game Theory Optimal) has been used a lot in poker recently. To clarify its meaning and application, the aim of this article is to define what it is, when to use it when playing, what strategies to apply for how to play GTO poker, for beginner and more advanced players!
Poker GTO
In poker, you can choose between two main winning strategies:
Exploitative play maximizes expected value (EV) by countering opponents' sub-optimal plays and weaker tendencies. Yes, playing this way opens you up to being exploited, but the weaker opponents you're targeting won't change their game to counteract this, allowing you to reap maximum profits over the long run.
GTO (Game-Theory Optimal): You try to play perfect poker, which forces your opponents to make mistakes (which is where almost all of your profit will be derived from). It mixes bluffs or semi-bluffs with value bets, clarifies bet sizes, and more.
GTO vs. Exploitative: Which is Better in Poker?
Before diving into GTO poker strategy, it's important to know which of these two play styles is more profitable for beginners and advanced players. The simple answer is probably both, but usually more exploitable.
Most players don't play GTO poker and can be exploited in their gameplay and strategy, allowing for more profits to be made using an exploitative approach. In fact, it’s only in some of the largest games at the highest stakes that GTO concepts are fully utilized and seen in practice, and even then, exploitative plays are still sometimes used.
Knowing, understanding, and applying GTO poker basics will create a solid foundation for your poker game. It's also important to understand GTO so you can deviate from it to maximize profits.
GTO Poker Strategy
According to Ed Miller's book "Poker's 1%," the most fundamental concept that only elite poker players understand is frequency, which could be in relation to cbets, bluffs, folds, calls, raises, etc.
GTO poker solvers (downloadable online software) give solutions for how to play optimally in any given spot and often recommend using mixed strategies based on select frequencies.
In a river situation, a solver may tell you to call 70% of the time and fold 30%. It may also suggest calling 50% of the time, folding 35% of the time, and raising 15% of the time (with a certain range of hands).
Frequencies are a fundamental and often unrecognized part of poker, but they run through these 5 GTO concepts.
1. Preflop ranges
To compensate for positional disadvantage, out-of-position players must open tighter hand ranges.
Premium starting hands aren't enough, though. Considering GTO poker ranges and principles, you want a good, balanced starting hand range from each position with at least some hands that can make a strong poker hand regardless of the flop texture (low, mid, high, disconnected, etc).
Below is a GTO preflop beginner poker chart for online 6-max play, showing which hand ranges one should open-raise with. Table positions are color-coded (see key below).
NOTE: For GTO play, it's advisable to use a mixed strategy for opening in the small blind, combining open-limps and open-raises for various hands. This cannot be illustrated with the color system used for the chart.
Choosing which hands to play is often a math problem, as discussed below.
Other preflop GTO poker charts include which hands to play after a raise, which to 3bet, etc. Solvers can help you decide which preflop hands to play (call, raise, re-raise, etc.).
2. Pot Odds
Always make +EV decisions that profit you as a poker player. Understanding pot odds (and equity) can help.
Postflop Pot Odds
Let’s say that we have JhTh on a board of 9h8h2s4c (open-ended straight-flush draw). We have $40 left and $50 in the pot. He has you covered and goes all-in. As calling or folding are our only options, playing GTO involves calculating whether a call is +EV or –EV. (The hand was empty.)
Any remaining heart, Queen, or 7 wins the hand. This means we can improve 15 of 46 unknown cards, or 32.6% of the time.
What if our opponent has a set? The 4h or 2h could give us a flush, but it could also give the villain a boat. If we reduce outs from 15 to 14.5, our equity would be 31.5%.
We must now calculate pot odds.
(bet/(our bet+pot)) = pot odds
= $50 / ($40 + $90)
= $40 / $130
= 30.7%
To make a profitable call, we need at least 30.7% equity. This is a profitable call as we have 31.5% equity (even if villain has a set). Yes, we will lose most of the time, but we will make a small profit in the long run, making a call correct.
Pot odds aren't just for draws, either. If an opponent bets 50% pot, you get 3 to 1 odds on a call, so you must win 25% of the time to be profitable. If your current hand has more than 25% equity against your opponent's perceived range, call.
Preflop Pot Odds
Preflop, you raise to 3bb and the button 3bets to 9bb. You must decide how to act. In situations like these, we can actually use pot odds to assist our decision-making.
This pot is:
(our open+3bet size+small blind+big blind)
(3bb+9bb+0.5bb+1bb)
= 13.5
This means we must call 6bb to win a pot of 13.5bb, which requires 30.7% equity against the 3bettor's range.
Three additional factors must be considered:
Being out of position on our opponent makes it harder to realize our hand's equity, as he can use his position to put us in tough spots. To profitably continue against villain's hand range, we should add 7% to our equity.
Implied Odds / Reverse Implied Odds: The ability to win or lose significantly more post-flop (than pre-flop) based on our remaining stack.
While statistics on 3bet stats can be gained with a large enough sample size (i.e. 8% 3bet stat from button), the numbers don't tell us which 8% of hands villain could be 3betting with. Both polarized and depolarized charts below show 8% of possible hands.
7.4% of hands are depolarized.
Polarized Hand range (7.54%):
Each hand range has different contents. We don't know if he 3bets some hands and calls or folds others.
Using an exploitable strategy can help you play a hand range correctly. The next GTO concept will make things easier.
3. Minimum Defense Frequency:
This concept refers to the % of our range we must continue with (by calling or raising) to avoid being exploited by our opponents. This concept is most often used off-table and is difficult to apply in-game.
These beginner GTO concepts will help your decision-making during a hand, especially against aggressive opponents.
MDF formula:
MDF = POT SIZE/(POT SIZE+BET SIZE)
Here's a poker GTO chart of common bet sizes and minimum defense frequency.
Take the number of hand combos in your starting hand range and use the MDF to determine which hands to continue with. Choose hands with the most playability and equity against your opponent's betting range.
Say you open-raise HJ and BB calls. Qh9h6c flop. Your opponent leads you for a half-pot bet. MDF suggests keeping 67% of our range.
Using the above starting hand chart, we can determine that the HJ opens 254 combos:
We must defend 67% of these hands, or 170 combos, according to MDF. Hands we should keep include:
Flush draws
Open-Ended Straight Draws
Gut-Shot Straight Draws
Overcards
Any Pair or better
So, our flop continuing range could be:
Some highlights:
Fours and fives have little chance of improving on the turn or river.
We only continue with AX hearts (with a flush draw) without a pair or better.
We'll also include 4 AJo combos, all of which have the Ace of hearts, and AcJh, which can block a backdoor nut flush combo.
Let's assume all these hands are called and the turn is blank (2 of spades). Opponent bets full-pot. MDF says we must defend 50% of our flop continuing range, or 85 of 170 combos, to be unexploitable. This strategy includes our best flush draws, straight draws, and made hands.
Here, we keep combining:
Nut flush draws
Pair + flush draws
GS + flush draws
Second Pair, Top Kicker+
One combo of JJ that doesn’t block the flush draw or backdoor flush draw.
On the river, we can fold our missed draws and keep our best made hands. When calling with weaker hands, consider blocker effects and card removal to avoid overcalling and decide which combos to continue.
4. Poker GTO Bet Sizing
To avoid being exploited, balance your bluffs and value bets. Your betting range depends on how much you bet (in relation to the pot). This concept only applies on the river, as draws (bluffs) on the flop and turn still have equity (and are therefore total bluffs).
On the flop, you want a 2:1 bluff-to-value-bet ratio. On the flop, there won't be as many made hands as on the river, and your bluffs will usually contain equity. The turn should have a "bluffing" ratio of 1:1. Use the chart below to determine GTO river bluff frequencies (relative to your bet size):
This chart relates to your opponent's pot odds. If you bet 50% pot, your opponent gets 3:1 odds and must win 25% of the time to call. Poker GTO theory suggests including 25% bluff combinations in your betting range so you're indifferent to your opponent calling or folding.
Best river bluffs don't block hands you want your opponent to have (or not have). For example, betting with missed Ace-high flush draws is often a mistake because you block a missed flush draw you want your opponent to have when bluffing on the river (meaning that it would subsequently be less likely he would have it, if you held two of the flush draw cards). Ace-high usually has some river showdown value.
If you had a 3-flush on the river and wanted to raise, you could bluff raise with AX combos holding the bluff suit Ace. Blocking the nut flush prevents your opponent from using that combo.
5. Bet Sizes and Frequency
GTO beginner strategies aren't just bluffs and value bets. They show how often and how much to bet in certain spots. Top players have benefited greatly from poker solvers, which we'll discuss next.
GTO Poker Software
In recent years, various poker GTO solvers have been released to help beginner, intermediate, and advanced players play balanced/GTO poker in various situations.
PokerSnowie and PioSolver are popular GTO and poker study programs.
While you can't compute players' hand ranges and what hands to bet or check with in real time, studying GTO play strategies with these programs will pay off. It will improve your poker thinking and understanding.
Solvers can help you balance ranges, choose optimal bet sizes, and master cbet frequencies.
GTO Poker Tournament
Late-stage tournaments have shorter stacks than cash games. In order to follow GTO poker guidelines, Nash charts have been created, tweaked, and used for many years (and also when to call, depending on what number of big blinds you have when you find yourself shortstacked).
The charts are for heads-up push/fold. In a multi-player game, the "pusher" chart can only be used if play is folded to you in the small blind. The "caller" chart can only be used if you're in the big blind and assumes a small blind "pusher" (with a much wider range than if a player in another position was open-shoving).
Divide the pusher chart's numbers by 2 to see which hand to use from the Button. Divide the original chart numbers by 4 to find the CO's pushing range. Some of the figures will be impossible to calculate accurately for the CO or positions to the right of the blinds because the chart's highest figure is "20+" big blinds, which is also used for a wide range of hands in the push chart.
Both of the GTO charts below are ideal for heads-up play, but exploitable HU shortstack strategies can lead to more +EV decisions against certain opponents. Following the charts will make your play GTO and unexploitable.
Poker pro Max Silver created the GTO push/fold software SnapShove. (It's accessible online at www.snapshove.com or as iOS or Android apps.)
Players can access GTO shove range examples in the full version. (You can customize the number of big blinds you have, your position, the size of the ante, and many other options.)
In Conclusion
Due to the constantly changing poker landscape, players are always improving their skills. Exploitable strategies often yield higher profit margins than GTO-based approaches, but knowing GTO beginner and advanced concepts can give you an edge for a few reasons.
It creates a solid gameplay base.
Having a baseline makes it easier to exploit certain villains.
You can avoid leveling wars with your opponents by making sound poker decisions based on GTO strategy.
It doesn't require assuming opponents' play styles.
Not results-oriented.
This is just the beginning of GTO and poker theory. Consider investing in the GTO poker solver software listed above to improve your game.
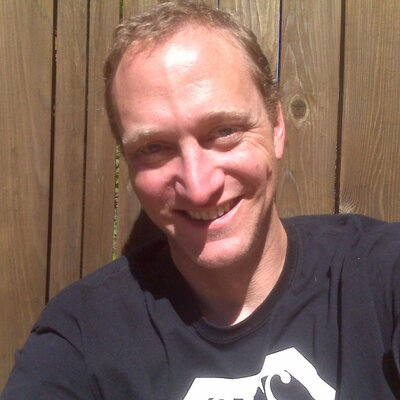
Bob Service
3 years ago
Did volcanic 'glasses' play a role in igniting early life?
Quenched lava may have aided in the formation of long RNA strands required by primitive life.
It took a long time for life to emerge. Microbes were present 3.7 billion years ago, just a few hundred million years after the 4.5-billion-year-old Earth had cooled enough to sustain biochemistry, according to fossils, and many scientists believe RNA was the genetic material for these first species. RNA, while not as complicated as DNA, would be difficult to forge into the lengthy strands required to transmit genetic information, raising the question of how it may have originated spontaneously.
Researchers may now have a solution. They demonstrate how basaltic glasses assist individual RNA letters, also known as nucleoside triphosphates, join into strands up to 200 letters long in lab studies. The glasses are formed when lava is quenched in air or water, or when melted rock generated by asteroid strikes cools rapidly, and they would have been plentiful in the early Earth's fire and brimstone.
The outcome has caused a schism among top origin-of-life scholars. "This appears to be a great story that finally explains how nucleoside triphosphates react with each other to create RNA strands," says Thomas Carell, a scientist at Munich's Ludwig Maximilians University. However, Harvard University's Jack Szostak, an RNA expert, says he won't believe the results until the study team thoroughly describes the RNA strands.
Researchers interested in the origins of life like the idea of a primordial "RNA universe" since the molecule can perform two different functions that are essential for life. It's made up of four chemical letters, just like DNA, and can carry genetic information. RNA, like proteins, can catalyze chemical reactions that are necessary for life.
However, RNA can cause headaches. No one has yet discovered a set of plausible primordial conditions that would cause hundreds of RNA letters—each of which is a complicated molecule—to join together into strands long enough to support the intricate chemistry required to kick-start evolution.
Basaltic glasses may have played a role, according to Stephen Mojzsis, a geologist at the University of Colorado, Boulder. They're high in metals like magnesium and iron, which help to trigger a variety of chemical reactions. "Basaltic glass was omnipresent on Earth at the time," he adds.
He provided the Foundation for Applied Molecular Evolution samples of five different basalt glasses. Each sample was ground into a fine powder, sanitized, and combined with a solution of nucleoside triphosphates by molecular biologist Elisa Biondi and her colleagues. The RNA letters were unable to link up without the presence of glass powder. However, when the molecules were mixed with the glass particles, they formed long strands of hundreds of letters, according to the researchers, who published their findings in Astrobiology this week. There was no need for heat or light. Biondi explains, "All we had to do was wait." After only a day, little RNA strands produced, yet the strands continued to grow for months. Jan Paek, a molecular biologist at Firebird Biomolecular Sciences, says, "The beauty of this approach is its simplicity." "Mix the components together, wait a few days, and look for RNA."
Nonetheless, the findings pose a slew of problems. One of the questions is how nucleoside triphosphates came to be in the first place. Recent study by Biondi's colleague Steven Benner suggests that the same basaltic glasses may have aided in the creation and stabilization of individual RNA letters.
The form of the lengthy RNA strands, according to Szostak, is a significant challenge. Enzymes in modern cells ensure that most RNAs form long linear chains. RNA letters, on the other hand, can bind in complicated branching sequences. Szostak wants the researchers to reveal what kind of RNA was produced by the basaltic glasses. "It irritates me that the authors made an intriguing initial finding but then chose to follow the hype rather than the research," Szostak says.
Biondi acknowledges that her team's experiment almost probably results in some RNA branching. She does acknowledge, however, that some branched RNAs are seen in species today, and that analogous structures may have existed before the origin of life. Other studies carried out by the study also confirmed the presence of lengthy strands with connections, indicating that they are most likely linear. "It's a healthy argument," says Dieter Braun, a Ludwig Maximilian University origin-of-life chemist. "It will set off the next series of tests."