More on Technology
Colin Faife
1 year ago
The brand-new USB Rubber Ducky is much riskier than before.
The brand-new USB Rubber Ducky is much riskier than before.
With its own programming language, the well-liked hacking tool may now pwn you.
With a vengeance, the USB Rubber Ducky is back.
This year's Def Con hacking conference saw the release of a new version of the well-liked hacking tool, and its author, Darren Kitchen, was on hand to explain it. We put a few of the new features to the test and discovered that the most recent version is riskier than ever.
WHAT IS IT?
The USB Rubber Ducky seems to the untrained eye to be an ordinary USB flash drive. However, when you connect it to a computer, the computer recognizes it as a USB keyboard and will accept keystroke commands from the device exactly like a person would type them in.
Kitchen explained to me, "It takes use of the trust model built in, where computers have been taught to trust a human, in that anything it types is trusted to the same degree as the user is trusted. And a computer is aware that clicks and keystrokes are how people generally connect with it.
Over ten years ago, the first Rubber Ducky was published, quickly becoming a hacker favorite (it was even featured in a Mr. Robot scene). Since then, there have been a number of small upgrades, but the most recent Rubber Ducky takes a giant step ahead with a number of new features that significantly increase its flexibility and capability.
WHERE IS ITS USE?
The options are nearly unlimited with the proper strategy.
The Rubber Ducky has already been used to launch attacks including making a phony Windows pop-up window to collect a user's login information or tricking Chrome into sending all saved passwords to an attacker's web server. However, these attacks lacked the adaptability to operate across platforms and had to be specifically designed for particular operating systems and software versions.
The nuances of DuckyScript 3.0 are described in a new manual.
The most recent Rubber Ducky seeks to get around these restrictions. The DuckyScript programming language, which is used to construct the commands that the Rubber Ducky will enter into a target machine, receives a significant improvement with it. DuckyScript 3.0 is a feature-rich language that allows users to write functions, store variables, and apply logic flow controls, in contrast to earlier versions that were primarily limited to scripting keystroke sequences (i.e., if this... then that).
This implies that, for instance, the new Ducky can check to see if it is hooked into a Windows or Mac computer and then conditionally run code specific to each one, or it can disable itself if it has been attached to the incorrect target. In order to provide a more human effect, it can also generate pseudorandom numbers and utilize them to add a configurable delay between keystrokes.
The ability to steal data from a target computer by encoding it in binary code and transferring it through the signals intended to instruct a keyboard when the CapsLock or NumLock LEDs should light up is perhaps its most astounding feature. By using this technique, a hacker may plug it in for a brief period of time, excuse themselves by saying, "Sorry, I think that USB drive is faulty," and then take it away with all the credentials stored on it.
HOW SERIOUS IS THE RISK?
In other words, it may be a significant one, but because physical device access is required, the majority of people aren't at risk of being a target.
The 500 or so new Rubber Duckies that Hak5 brought to Def Con, according to Kitchen, were his company's most popular item at the convention, and they were all gone on the first day. It's safe to suppose that hundreds of hackers already possess one, and demand is likely to persist for some time.
Additionally, it has an online development toolkit that can be used to create attack payloads, compile them, and then load them onto the target device. A "payload hub" part of the website makes it simple for hackers to share what they've generated, and the Hak5 Discord is also busy with conversation and helpful advice. This makes it simple for users of the product to connect with a larger community.
It's too expensive for most individuals to distribute in volume, so unless your favorite cafe is renowned for being a hangout among vulnerable targets, it's doubtful that someone will leave a few of them there. To that end, if you intend to plug in a USB device that you discovered outside in a public area, pause to consider your decision.
WOULD IT WORK FOR ME?
Although the device is quite straightforward to use, there are a few things that could cause you trouble if you have no prior expertise writing or debugging code. For a while, during testing on a Mac, I was unable to get the Ducky to press the F4 key to activate the launchpad, but after forcing it to identify itself using an alternative Apple keyboard device ID, the problem was resolved.
From there, I was able to create a script that, when the Ducky was plugged in, would instantly run Chrome, open a new browser tab, and then immediately close it once more without requiring any action from the laptop user. Not bad for only a few hours of testing, and something that could be readily changed to perform duties other than reading technology news.

M.G. Siegler
1 year ago
G3nerative
Generative AI hype: some thoughts
The sudden surge in "generative AI" startups and projects feels like the inverse of the recent "web3" boom. Both came from hyped-up pots. But while web3 hyped idealistic tech and an easy way to make money, generative AI hypes unsettling tech and questions whether it can be used to make money.
Web3 is technology looking for problems to solve, while generative AI is technology creating almost too many solutions. Web3 has been evangelists trying to solve old problems with new technology. As Generative AI evolves, users are resolving old problems in stunning new ways.
It's a jab at web3, but it's true. Web3's hype, including crypto, was unhealthy. Always expected a tech crash and shakeout. Tech that won't look like "web3" but will enhance "web2"
But that doesn't mean AI hype is healthy. There'll be plenty of bullshit here, too. As moths to a flame, hype attracts charlatans. Again, the difference is the different starting point. People want to use it. Try it.
With the beta launch of Dall-E 2 earlier this year, a new class of consumer product took off. Midjourney followed suit (despite having to jump through the Discord server hoops). Twelve more generative art projects. Lensa, Prisma Labs' generative AI self-portrait project, may have topped the hype (a startup which has actually been going after this general space for quite a while). This week, ChatGPT went off-topic.
This has a "fake-it-till-you-make-it" vibe. We give these projects too much credit because they create easy illusions. This also unlocks new forms of creativity. And faith in new possibilities.
As a user, it's thrilling. We're just getting started. These projects are not only fun to play with, but each week brings a new breakthrough. As an investor, it's all happening so fast, with so much hype (and ethical and societal questions), that no one knows how it will turn out. Web3's demand won't be the issue. Too much demand may cause servers to melt down, sending costs soaring. Companies will try to mix rapidly evolving tech to meet user demand and create businesses. Frustratingly difficult.
Anyway, I wanted an excuse to post some Lensa selfies.
These are really weird. I recognize them as me or a version of me, but I have no memory of them being taken. It's surreal, out-of-body. Uncanny Valley.

Nikhil Vemu
1 year ago
7 Mac Tips You Never Knew You Needed
Unleash the power of the Option key ⌥
#1 Open a link in the Private tab first.
Previously, if I needed to open a Safari link in a private window, I would:
copied the URL with the right click command,
choose File > New Private Window to open a private window, and
clicked return after pasting the URL.
I've found a more straightforward way.
Right-clicking a link shows this, right?
Hold option (⌥) for:
Click Open Link in New Private Window while holding.
Finished!
#2. Instead of searching for specific characters, try this
You may use unicode for business or school. Most people Google them when they need them.
That is lengthy!
You can type some special characters just by pressing ⌥ and a key.
For instance
• ⌥+2 -> ™ (Trademark)
• ⌥+0 -> ° (Degree)
• ⌥+G -> © (Copyright)
• ⌥+= -> ≠ (Not equal to)
• ⌥+< -> ≤ (Less than or equal to)
• ⌥+> -> ≥ (Greater then or equal to)
• ⌥+/ -> ÷ (Different symbol for division)
#3 Activate Do Not Disturb silently.
Do Not Disturb when sharing my screen is awkward for me (because people may think Im trying to hide some secret notifications).
Here's another method.
Hold ⌥ and click on Time (at the extreme right on the menu-bar).
Now, DND is activated (secretly!). To turn it off, do it again.
Note: This works only for DND focus.
#4. Resize a window starting from its center
Although this is rarely useful, it is still a hidden trick.
When you resize a window, the opposite edge or corner is used as the pivot, right?
However, if you want to resize it with its center as the pivot, hold while doing so.
#5. Yes, Cut-Paste is available on Macs as well (though it is slightly different).
I call it copy-move rather than cut-paste. This is how it works.
Carry it out.
Choose a file (by clicking on it), then copy it (⌘+C).
Go to a new location on your Mac. Do you use ⌘+V to paste it? However, to move it, press ⌘+⌥+V.
This removes the file from its original location and copies it here. And it works exactly like cut-and-paste on Windows.
#6. Instantly expand all folders
Set your Mac's folders to List view.
Assume you have one folder with multiple subfolders, each of which contains multiple files. And you wanted to look at every single file that was over there.
How would you do?
You're used to clicking the ⌄ glyph near the folder and each subfolder to expand them all, right? Instead, hold down ⌥ while clicking ⌄ on the parent folder.
This is what happens next.
Everything expands.
View/Copy a file's path as an added bonus
If you want to see the path of a file in Finder, select it and hold ⌥, and you'll see it at the bottom for a moment.
To copy its path, right-click on the folder and hold down ⌥ to see this
Click on Copy <"folder name"> as Pathname
to do it.
#7 "Save As"
I was irritated by the lack of "Save As" in Pages when I first got a Mac (after 15 years of being a Windows guy).
It was necessary for me to save the file as a new file, in a different location, with a different name, or both.
Unfortunately, I couldn't do it on a Mac.
However, I recently discovered that it appears when you hold ⌥ when in the File menu.
Yay!
You might also like
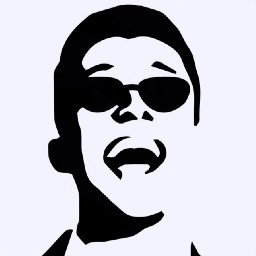
Joseph Mavericks
1 year ago
You Don't Have to Spend $250 on TikTok Ads Because I Did
900K impressions, 8K clicks, and $$$ orders…
I recently started dropshipping. Now that I own my business and can charge it as a business expense, it feels less like money wasted if it doesn't work. I also made t-shirts to sell. I intended to open a t-shirt store and had many designs on a hard drive. I read that Tiktok advertising had a high conversion rate and low cost because they were new. According to many, the advertising' cost/efficiency ratio would plummet and become as bad as Google or Facebook Ads. Now felt like the moment to try Tiktok marketing and dropshipping. I work in marketing for a SaaS firm and have seen how poorly ads perform. I wanted to try it alone.
I set up $250 and ran advertising for a week. Before that, I made my own products, store, and marketing. In this post, I'll show you my process and results.
Setting up the store
Dropshipping is a sort of retail business in which the manufacturer ships the product directly to the client through an online platform maintained by a seller. The seller takes orders but has no stock. The manufacturer handles all orders. This no-stock concept increases profitability and flexibility.
In my situation, I used previous t-shirt designs to make my own product. I didn't want to handle order fulfillment logistics, so I looked for a way to print my designs on demand, ship them, and handle order tracking/returns automatically. So I found Printful.
I needed to connect my backend and supplier to a storefront so visitors could buy. 99% of dropshippers use Shopify, but I didn't want to master the difficult application. I wanted a one-day project. I'd previously worked with Big Cartel, so I chose them.
Big Cartel doesn't collect commissions on sales, simply a monthly flat price ($9.99 to $19.99 depending on your plan).
After opening a Big Cartel account, I uploaded 21 designs and product shots, then synced each product with Printful.
Developing the ads
I mocked up my designs on cool people photographs from placeit.net, a great tool for creating product visuals when you don't have a studio, camera gear, or models to wear your t-shirts.
I opened an account on the website and had advertising visuals within 2 hours.
Because my designs are simple (black design on white t-shirt), I chose happy, stylish people on plain-colored backdrops. After that, I had to develop an animated slideshow.
Because I'm a graphic designer, I chose to use Adobe Premiere to create animated Tiktok advertising.
Premiere is a fancy video editing application used for more than advertisements. Premiere is used to edit movies, not social media marketing. I wanted this experiment to be quick, so I got 3 social media ad templates from motionarray.com and threw my visuals in. All the transitions and animations were pre-made in the files, so it only took a few hours to compile. The result:
I downloaded 3 different soundtracks for the videos to determine which would convert best.
After that, I opened a Tiktok business account, uploaded my films, and inserted ad info. They went live within one hour.
The (poor) outcomes
As a European company, I couldn't deliver ads in the US. All of my advertisements' material (title, description, and call to action) was in English, hence they continued getting rejected in Europe for countries that didn't speak English. There are a lot of them:
I lost a lot of quality traffic, but I felt that if the images were engaging, people would check out the store and buy my t-shirts. I was wrong.
51,071 impressions on Day 1. 0 orders after 411 clicks
114,053 impressions on Day 2. 1.004 clicks and no orders
Day 3: 987 clicks, 103,685 impressions, and 0 orders
101,437 impressions on Day 4. 0 orders after 963 clicks
115,053 impressions on Day 5. 1,050 clicks and no purchases
125,799 impressions on day 6. 1,184 clicks, no purchases
115,547 impressions on Day 7. 1,050 clicks and no purchases
121,456 impressions on day 8. 1,083 clicks, no purchases
47,586 impressions on Day 9. 419 Clicks. No orders
My overall conversion rate for video advertisements was 0.9%. TikTok's paid ad formats all result in strong engagement rates (ads average 3% to 12% CTR to site), therefore a 1 to 2% CTR should have been doable.
My one-week experiment yielded 8,151 ad clicks but no sales. Even if 0.1% of those clicks converted, I should have made 8 sales. Even companies with horrible web marketing would get one download or trial sign-up for every 8,151 clicks. I knew that because my advertising were in English, I had no impressions in the main EU markets (France, Spain, Italy, Germany), and that this impacted my conversion potential. I still couldn't believe my numbers.
I dug into the statistics and found that Tiktok's stats didn't match my store traffic data.
Looking more closely at the numbers
My ads were approved on April 26 but didn't appear until April 27. My store dashboard showed 440 visitors but 1,004 clicks on Tiktok. This happens often while tracking campaign results since different platforms handle comparable user activities (click, view) differently. In online marketing, residual data won't always match across tools.
My data gap was too large. Even if half of the 1,004 persons who clicked closed their browser or left before the store site loaded, I would have gained 502 visitors. The significant difference between Tiktok clicks and Big Cartel store visits made me suspicious. It happened all week:
Day 1: 440 store visits and 1004 ad clicks
Day 2: 482 store visits, 987 ad clicks
3rd day: 963 hits on ads, 452 store visits
443 store visits and 1,050 ad clicks on day 4.
Day 5: 459 store visits and 1,184 ad clicks
Day 6: 430 store visits and 1,050 ad clicks
Day 7: 409 store visits and 1,031 ad clicks
Day 8: 166 store visits and 418 ad clicks
The disparity wasn't related to residual data or data processing. The disparity between visits and clicks looked regular, but I couldn't explain it.
After the campaign concluded, I discovered all my creative assets (the videos) had a 0% CTR and a $0 expenditure in a separate dashboard. Whether it's a dashboard reporting issue or a budget allocation bug, online marketers shouldn't see this.
Tiktok can present any stats they want on their dashboard, just like any other platform that runs advertisements to promote content to its users. I can't verify that 895,687 individuals saw and clicked on my ad. I invested $200 for what appears to be around 900K impressions, which is an excellent ROI. No one bought a t-shirt, even an unattractive one, out of 900K people?
Would I do it again?
Nope. Whether I didn't make sales because Tiktok inflated the dashboard numbers or because I'm horrible at producing advertising and items that sell, I’ll stick to writing content and making videos. If setting up a business and ads in a few days was all it took to make money online, everyone would do it.
Video advertisements and dropshipping aren't dead. As long as the internet exists, people will click ads and buy stuff. Converting ads and selling stuff takes a lot of work, and I want to focus on other things.
I had always wanted to try dropshipping and I’m happy I did, I just won’t stick to it because that’s not something I’m interested in getting better at.
If I want to sell t-shirts again, I'll avoid Tiktok advertisements and find another route.

Al Anany
1 year ago
Because of this covert investment that Bezos made, Amazon became what it is today.
He kept it under wraps for years until he legally couldn’t.
His shirt is incomplete. I can’t stop thinking about this…
Actually, ignore the article. Look at it. JUST LOOK at it… It’s quite disturbing, isn’t it?
Ughh…
Me: “Hey, what up?” Friend: “All good, watching lord of the rings on amazon prime video.” Me: “Oh, do you know how Amazon grew and became famous?” Friend: “Geek alert…Can I just watch in peace?” Me: “But… Bezos?” Friend: “Let it go, just let it go…”
I can question you, the reader, and start answering instantly without his consent. This far.
Reader, how did Amazon succeed? You'll say, Of course, it was an internet bookstore, then it sold everything.
Mistaken. They moved from zero to one because of this. How did they get from one to thousand? AWS-some. Understand? It's geeky and lame. If not, I'll explain my geekiness.
Over an extended period of time, Amazon was not profitable.
Business basics. You want customers if you own a bakery, right?
Well, 100 clients per day order $5 cheesecakes (because cheesecakes are awesome.)
$5 x 100 consumers x 30 days Equals $15,000 monthly revenue. You proudly work here.
Now you have to pay the barista (unless ChatGPT is doing it haha? Nope..)
The barista is requesting $5000 a month.
Each cheesecake costs the cheesecake maker $2.5 ($2.5 × 100 x 30 = $7500).
The monthly cost of running your bakery, including power, is about $5000.
Assume no extra charges. Your operating costs are $17,500.
Just $15,000? You have income but no profit. You might make money selling coffee with your cheesecake next month.
Is losing money bad? You're broke. Losing money. It's bad for financial statements.
It's almost a business ultimatum. Most startups fail. Amazon took nine years.
I'm reading Amazon Unbound: Jeff Bezos and the Creation of a Global Empire to comprehend how a company has a $1 trillion market cap.
Many things made Amazon big. The book claims that Bezos and Amazon kept a specific product secret for a long period.
Clouds above the bald head.
In 2006, Bezos started a cloud computing initiative. They believed many firms like Snapchat would pay for reliable servers.
In 2006, cloud computing was not what it is today. I'll simplify. 2006 had no iPhone.
Bezos invested in Amazon Web Services (AWS) without disclosing its revenue. That's permitted till a certain degree.
Google and Microsoft would realize Amazon is heavily investing in this market and worry.
Bezos anticipated high demand for this product. Microsoft built its cloud in 2010, and Google in 2008.
If you managed Google or Microsoft, you wouldn't know how much Amazon makes from their cloud computing service. It's enough. Yet, Amazon is an internet store, so they'll focus on that.
All but Bezos were wrong.
Time to come clean now.
They revealed AWS revenue in 2015. Two things were apparent:
Bezos made the proper decision to bet on the cloud and keep it a secret.
In this race, Amazon is in the lead.
They continued. Let me list some AWS users today.
Netflix
Airbnb
Twitch
More. Amazon was unprofitable for nine years, remember? This article's main graph.
AWS accounted for 74% of Amazon's profit in 2021. This 74% might not exist if they hadn't invested in AWS.
Bring this with you home.
Amazon predated AWS. Yet, it helped the giant reach $1 trillion. Bezos' secrecy? Perhaps, until a time machine is invented (they might host the time machine software on AWS, though.)
Without AWS, Amazon would have been profitable but unimpressive. They may have invested in anything else that would have returned more (like crypto? No? Ok.)
Bezos has business flaws. His success. His failures include:
introducing the Fire Phone and suffering a $170 million loss.
Amazon's failure in China In 2011, Amazon had a about 15% market share in China. 2019 saw a decrease of about 1%.
not offering a higher price to persuade the creator of Netflix to sell the company to him. He offered a rather reasonable $15 million in his proposal. But what if he had offered $30 million instead (Amazon had over $100 million in revenue at the time)? He might have owned Netflix, which has a $156 billion market valuation (and saved billions rather than invest in Amazon Prime Video).
Some he could control. Some were uncontrollable. Nonetheless, every action he made in the foregoing circumstances led him to invest in AWS.

Nojus Tumenas
1 year ago
NASA: Strange Betelgeuse Explosion Just Took Place
Orion's red supergiant Betelgeuse erupted. This is astronomers' most magnificent occurrence.
Betelgeuse, a supergiant star in Orion, garnered attention in 2019 for its peculiar appearance. It continued to dim in 2020.
The star was previously thought to explode as a supernova. Studying the event has revealed what happened to Betelgeuse since it happened.
Astronomers saw that the star released a large amount of material, causing it to lose a section of its surface.
They have never seen anything like this and are unsure what caused the star to release so much material.
According to Harvard-Smithsonian Center for Astrophysics astrophysicist Andrea Dupre, astronomers' data reveals an unexplained mystery.
They say it's a new technique to examine star evolution. The James Webb telescope revealed the star's surface features.
Corona flares are stellar mass ejections. These eruptions change the Sun's outer atmosphere.
This could affect power grids and satellite communications if it hits Earth.
Betelgeuse's flare ejected four times more material than the Sun's corona flare.
Astronomers have monitored star rhythms for 50 years. They've seen its dimming and brightening cycle start, stop, and repeat.
Monitoring Betelgeuse's pulse revealed the eruption's power.
Dupre believes the star's convection cells are still amplifying the blast's effects, comparing it to an imbalanced washing machine tub.
The star's outer layer has returned to normal, Hubble data shows. The photosphere slowly rebuilds its springy surface.
Dupre noted the star's unusual behavior. For instance, it’s causing its interior to bounce.
This suggests that the mass ejections that caused the star's surface to lose mass were two separate processes.
Researchers hope to better understand star mass ejection with the James Webb Space Telescope.