More on NFTs & Art

Anton Franzen
2 years ago
This is the driving force for my use of NFTs, which will completely transform the world.
Its not a fuc*ing fad.
It's not about boring monkeys or photos as nfts; that's just what's been pushed up and made a lot of money. The technology underlying those ridiculous nft photos will one day prove your house and automobile ownership and tell you where your banana came from. Are you ready for web3? Soar!
People don't realize that absolutely anything can and will be part of the blockchain and smart contracts, making them even better. I'll tell you a secret: it will and is happening.
Why?
Why is something blockchain-based a good idea? So let’s speak about cars!
So a new Tesla car is manufactured, and when you buy it, it is bound to an NFT on the blockchain that proves current ownership. The NFT in the smart contract can contain some data about the current owner of the car and some data about the car's status, such as the number of miles driven, the car's overall quality, and so on, as well as a reference to a digital document bound to the NFT that has more information.
Now, 40 years from now, if you want to buy a used automobile, you can scan the car's serial number to view its NFT and see all of its history, each owner, how long they owned it, if it had damages, and more. Since it's on the blockchain, it can't be tampered with.
When you're ready to buy it, the owner posts it for sale, you buy it, and it's sent to your wallet. 5 seconds to change owner, 100% safe and verifiable.
Incorporate insurance logic into the car contract. If you crashed, your car's smart contract would take money from your insurance contract and deposit it in an insurance company wallet.
It's limitless. Your funds may be used by investors to provide insurance as they profit from everyone's investments.
Or suppose all car owners in a country deposit a fixed amount of money into an insurance smart contract that promises if something happens, we'll take care of it. It could be as little as $100-$500 per year, and in a country with 10 million people, maybe 3 million would do that, which would be $500 000 000 in that smart contract and it would be used by the insurance company to invest in assets or take a cut, literally endless possibilities.
Instead of $300 per month, you may pay $300 per year to be covered if something goes wrong, and that may include multiple insurances.
What about your grocery store banana, though?
Yes that too.
You can scan a banana to learn its complete history. You'll be able to see where it was cultivated, every middleman in the supply chain, and hopefully the banana's quality, farm, and ingredients used.
If you want locally decent bananas, you can only buy them, offering you transparency and options. I believe it will be an online marketplace where farmers publish their farms and products for trust and transparency. You might also buy bananas from the farmer.
And? Food security to finish the article. If an order of bananas included a toxin, you could easily track down every banana from the same origin and supply chain and uncover the root cause. This is a tremendous thing that will save lives and have a big impact; did you realize that 1 in 6 Americans gets poisoned by food every year? This could lower the number.
To summarize:
Smart contracts can issue nfts as proof of ownership and include functionality.

Boris Müller
2 years ago
Why Do Websites Have the Same Design?
My kids redesigned the internet because it lacks inventiveness.
Internet today is bland. Everything is generic: fonts, layouts, pages, and visual language. Microtypography is messy.
Web design today seems dictated by technical and ideological constraints rather than creativity and ideas. Text and graphics are in containers on every page. All design is assumed.
Ironically, web technologies can design a lot. We can execute most designs. We make shocking, evocative websites. Experimental typography, generating graphics, and interactive experiences are possible.
Even designer websites use containers in containers. Dribbble and Behance, the two most popular creative websites, are boring. Lead image.
How did this happen?
Several reasons. WordPress and other blogging platforms use templates. These frameworks build web pages by combining graphics, headlines, body content, and videos. Not designs, templates. These rules combine related data types. These platforms don't let users customize pages beyond the template. You filled the template.
Templates are content-neutral. Thus, the issue.
Form should reflect and shape content, which is a design principle. Separating them produces content containers. Templates have no design value.
One of the fundamental principles of design is a deep and meaningful connection between form and content.
Web design lacks imagination for many reasons. Most are pragmatic and economic. Page design takes time. Large websites lack the resources to create a page from scratch due to the speed of internet news and the frequency of new items. HTML, JavaScript, and CSS continue to challenge web designers. Web design can't match desktop publishing's straightforward operations.
Designers may also be lazy. Mobile-first, generic, framework-driven development tends to ignore web page visual and contextual integrity.
How can we overcome this? How might expressive and avant-garde websites look today?
Rediscovering the past helps design the future.
'90s-era web design
At the University of the Arts Bremen's research and development group, I created my first website 23 years ago. Web design was trendy. Young web. Pages inspired me.
We struggled with HTML in the mid-1990s. Arial, Times, and Verdana were the only web-safe fonts. Anything exciting required table layouts, monospaced fonts, or GIFs. HTML was originally content-driven, thus we had to work against it to create a page.
Experimental typography was booming. Designers challenged the established quo from Jan Tschichold's Die Neue Typographie in the twenties to April Greiman's computer-driven layouts in the eighties. By the mid-1990s, an uncommon confluence of technological and cultural breakthroughs enabled radical graphic design. Irma Boom, David Carson, Paula Scher, Neville Brody, and others showed it.
Early web pages were dull compared to graphic design's aesthetic explosion. The Web Design Museum shows this.
Nobody knew how to conduct browser-based graphic design. Web page design was undefined. No standards. No CMS (nearly), CSS, JS, video, animation.
Now is as good a time as any to challenge the internet’s visual conformity.
In 2018, everything is browser-based. Massive layouts to micro-typography, animation, and video. How do we use these great possibilities? Containerized containers. JavaScript-contaminated mobile-first pages. Visually uniform templates. Web design 23 years later would disappoint my younger self.
Our imagination, not technology, restricts web design. We're too conformist to aesthetics, economics, and expectations.
Crisis generates opportunity. Challenge online visual conformity now. I'm too old and bourgeois to develop a radical, experimental, and cutting-edge website. I can ask my students.
I taught web design at the Potsdam Interface Design Programme in 2017. Each team has to redesign a website. Create expressive, inventive visual experiences on the browser. Create with contemporary web technologies. Avoid usability, readability, and flexibility concerns. Act. Ignore Erwartungskonformität.
The class outcome pleased me. This overview page shows all results. Four diverse projects address the challenge.
1. ZKM by Frederic Haase and Jonas Köpfer
Frederic and Jonas began their experiments on the ZKM website. The ZKM is Germany's leading media art exhibition location, but its website remains conventional. It's useful but not avant-garde like the shows' art.
Frederic and Jonas designed the ZKM site's concept, aesthetic language, and technical configuration to reflect the museum's progressive approach. A generative design engine generates new layouts for each page load.
ZKM redesign.
2. Streem by Daria Thies, Bela Kurek, and Lucas Vogel
Street art magazine Streem. It promotes new artists and societal topics. Streem includes artwork, painting, photography, design, writing, and journalism. Daria, Bela, and Lucas used these influences to develop a conceptual metropolis. They designed four neighborhoods to reflect magazine sections for their prototype. For a legible city, they use powerful illustrative styles and spatial typography.
Streem makeover.
3. Medium by Amelie Kirchmeyer and Fabian Schultz
Amelie and Fabian structured. Instead of developing a form for a tale, they dissolved a web page into semantic, syntactical, and statistical aspects. HTML's flexibility was their goal. They broke Medium posts into experimental typographic space.
Medium revamp.
4. Hacker News by Fabian Dinklage and Florian Zia
Florian and Fabian made Hacker News interactive. The social networking site aggregates computer science and IT news. Its voting and debate features are extensive despite its simple style. Fabian and Florian transformed the structure into a typographic timeline and network area. News and comments sequence and connect the visuals. To read Hacker News, they connected their design to the API. Hacker News makeover.
Communication is not legibility, said Carson. Apply this to web design today. Modern websites must be legible, usable, responsive, and accessible. They shouldn't limit its visual palette. Visual and human-centered design are not stereotypes.
I want radical, generative, evocative, insightful, adequate, content-specific, and intelligent site design. I want to rediscover web design experimentation. More surprises please. I hope the web will appear different in 23 years.
Update: this essay has sparked a lively discussion! I wrote a brief response to the debate's most common points: Creativity vs. Usability

xuanling11
2 years ago
Reddit NFT Achievement
Reddit's NFT market is alive and well.
NFT owners outnumber OpenSea on Reddit.
Reddit NFTs flip in OpenSea in days:
Fast-selling.
NFT sales will make Reddit's current communities more engaged.
I don't think NFTs will affect existing groups, but they will build hype for people to acquire them.
The first season of Collectibles is unique, but many missed the first season.
Second-season NFTs are less likely to be sold for a higher price than first-season ones.
If you use Reddit, it's fun to own NFTs.
You might also like
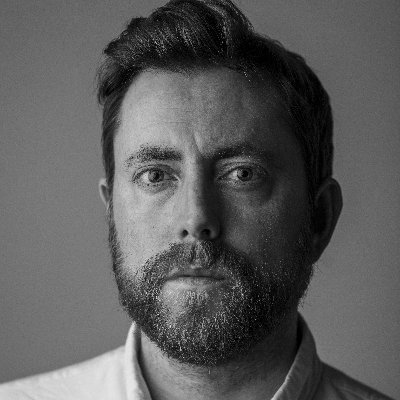
Liam Vaughan
2 years ago
Investors can bet big on almost anything on a new prediction market.
Kalshi allows five-figure bets on the Grammys, the next Covid wave, and future SEC commissioners. Worst-case scenario
On Election Day 2020, two young entrepreneurs received a call from the CFTC chairman. Luana Lopes Lara and Tarek Mansour spent 18 months trying to start a new type of financial exchange. Instead of betting on stock prices or commodity futures, people could trade instruments tied to real-world events, such as legislation, the weather, or the Oscar winner.
Heath Tarbert, a Trump appointee, shouted "Congratulations." "You're competing with 1840s-era markets. I'm sure you'll become a powerhouse too."
Companies had tried to introduce similar event markets in the US for years, but Tarbert's agency, the CFTC, said no, arguing they were gambling and prone to cheating. Now the agency has reversed course, approving two 24-year-olds who will have first-mover advantage in what could become a huge new asset class. Kalshi Inc. raised $30 million from venture capitalists within weeks of Tarbert's call, his representative says. Mansour, 26, believes this will be bigger than crypto.
Anyone who's read The Wisdom of Crowds knows prediction markets' potential. Well-designed markets can help draw out knowledge from disparate groups, and research shows that when money is at stake, people make better predictions. Lopes Lara calls it a "bullshit tax." That's why Google, Microsoft, and even the US Department of Defense use prediction markets internally to guide decisions, and why university-linked political betting sites like PredictIt sometimes outperform polls.
Regulators feared Wall Street-scale trading would encourage investors to manipulate reality. If the stakes are high enough, traders could pressure congressional staffers to stall a bill or bet on whether Kanye West's new album will drop this week. When Lopes Lara and Mansour pitched the CFTC, senior regulators raised these issues. Politically appointed commissioners overruled their concerns, and one later joined Kalshi's board.
Will Kanye’s new album come out next week? Yes or no?
Kalshi's victory was due more to lobbying and legal wrangling than to Silicon Valley-style innovation. Lopes Lara and Mansour didn't invent anything; they changed a well-established concept's governance. The result could usher in a new era of market-based enlightenment or push Wall Street's destructive tendencies into the real world.
If Kalshi's founders lacked experience to bolster their CFTC application, they had comical youth success. Lopes Lara studied ballet at the Brazilian Bolshoi before coming to the US. Mansour won France's math Olympiad. They bonded over their work ethic in an MIT computer science class.
Lopes Lara had the idea for Kalshi while interning at a New York hedge fund. When the traders around her weren't working, she noticed they were betting on the news: Would Apple hit a trillion dollars? Kylie Jenner? "It was anything," she says.
Are mortgage rates going up? Yes or no?
Mansour saw the business potential when Lopes Lara suggested it. He interned at Goldman Sachs Group Inc., helping investors prepare for the UK leaving the EU. Goldman sold clients complex stock-and-derivative combinations. As he discussed it with Lopes Lara, they agreed that investors should hedge their risk by betting on Brexit itself rather than an imperfect proxy.
Lopes Lara and Mansour hypothesized how a marketplace might work. They settled on a "event contract," a binary-outcome instrument like "Will inflation hit 5% by the end of the month?" The contract would settle at $1 (if the event happened) or zero (if it didn't), but its price would fluctuate based on market sentiment. After a good debate, a politician's election odds may rise from 50 to 55. Kalshi would charge a commission on every trade and sell data to traders, political campaigns, businesses, and others.
In October 2018, five months after graduation, the pair flew to California to compete in a hackathon for wannabe tech founders organized by the Silicon Valley incubator Y Combinator. They built a website in a day and a night and presented it to entrepreneurs the next day. Their prototype barely worked, but they won a three-month mentorship program and $150,000. Michael Seibel, managing director of Y Combinator, said of their idea, "I had to take a chance!"
Will there be another moon landing by 2025?
Seibel's skepticism was rooted in America's historical wariness of gambling. Roulette, poker, and other online casino games are largely illegal, and sports betting was only legal in a few states until May 2018. Kalshi as a risk-hedging platform rather than a bookmaker seemed like a good idea, but convincing the CFTC wouldn't be easy. In 2012, the CFTC said trading on politics had no "economic purpose" and was "contrary to the public interest."
Lopes Lara and Mansour cold-called 60 Googled lawyers during their time at Y Combinator. Everyone advised quitting. Mansour recalls the pain. Jeff Bandman, a former CFTC official, helped them navigate the agency and its characters.
When they weren’t busy trying to recruit lawyers, Lopes Lara and Mansour were meeting early-stage investors. Alfred Lin of Sequoia Capital Operations LLC backed Airbnb, DoorDash, and Uber Technologies. Lin told the founders their idea could capitalize on retail trading and challenge how the financial world manages risk. "Come back with regulatory approval," he said.
In the US, even small bets on most events were once illegal. Under the Commodity Exchange Act, the CFTC can stop exchanges from listing contracts relating to "terrorism, assassination, war" and "gaming" if they are "contrary to the public interest," which was often the case.
Will subway ridership return to normal? Yes or no?
In 1988, as academic interest in the field grew, the agency allowed the University of Iowa to set up a prediction market for research purposes, as long as it didn't make a profit or advertise and limited bets to $500. PredictIt, the biggest and best-known political betting platform in the US, also got an exemption thanks to an association with Victoria University of Wellington in New Zealand. Today, it's a sprawling marketplace with its own subculture and lingo. PredictIt users call it "Rules Cuck Panther" when they lose on a technicality. Major news outlets cite PredictIt's odds on Discord and the Star Spangled Gamblers podcast.
CFTC limits PredictIt bets to $850. To keep traders happy, PredictIt will often run multiple variations of the same question, listing separate contracts for two dozen Democratic primary candidates, for example. A trader could have more than $10,000 riding on a single outcome. Some of the site's traders are current or former campaign staffers who can answer questions like "How many tweets will Donald Trump post from Nov. 20 to 27?" and "When will Anthony Scaramucci's role as White House communications director end?"
According to PredictIt co-founder John Phillips, politicians help explain the site's accuracy. "Prediction markets work well and are accurate because they attract people with superior information," he said in a 2016 podcast. “In the financial stock market, it’s called inside information.”
Will Build Back Better pass? Yes or no?
Trading on nonpublic information is illegal outside of academia, which presented a dilemma for Lopes Lara and Mansour. Kalshi's forecasts needed to be accurate. Kalshi must eliminate insider trading as a regulated entity. Lopes Lara and Mansour wanted to build a high-stakes PredictIt without the anarchy or blurred legal lines—a "New York Stock Exchange for Events." First, they had to convince regulators event trading was safe.
When Lopes Lara and Mansour approached the CFTC in the spring of 2019, some officials in the Division of Market Oversight were skeptical, according to interviews with people involved in the process. For all Kalshi's talk of revolutionizing finance, this was just a turbocharged version of something that had been rejected before.
The DMO couldn't see the big picture. The staff review was supposed to ensure Kalshi could complete a checklist, "23 Core Principles of a Designated Contract Market," which included keeping good records and having enough money. The five commissioners decide. With Trump as president, three of them were ideologically pro-market.
Lopes Lara, Mansour, and their lawyer Bandman, an ex-CFTC official, answered the DMO's questions while lobbying the commissioners on Zoom about the potential of event markets to mitigate risks and make better decisions. Before each meeting, they would write a script and memorize it word for word.
Will student debt be forgiven? Yes or no?
Several prediction markets that hadn't sought regulatory approval bolstered Kalshi's case. Polymarket let customers bet hundreds of thousands of dollars anonymously using cryptocurrencies, making it hard to track. Augur, which facilitates private wagers between parties using blockchain, couldn't regulate bets and hadn't stopped users from betting on assassinations. Kalshi, by comparison, argued it was doing everything right. (The CFTC fined Polymarket $1.4 million for operating an unlicensed exchange in January 2022. Polymarket says it's now compliant and excited to pioneer smart contract-based financial solutions with regulators.
Kalshi was approved unanimously despite some DMO members' concerns about event contracts' riskiness. "Once they check all the boxes, they're in," says a CFTC insider.
Three months after CFTC approval, Kalshi announced funding from Sequoia, Charles Schwab, and Henry Kravis. Sequoia's Lin, who joined the board, said Tarek, Luana, and team created a new way to invest and engage with the world.
The CFTC hadn't asked what markets the exchange planned to run since. After approval, Lopes Lara and Mansour had the momentum. Kalshi's March list of 30 proposed contracts caused chaos at the DMO. The division handles exchanges that create two or three new markets a year. Kalshi’s business model called for new ones practically every day.
Uncontroversial proposals included weather and GDP questions. Others, on the initial list and later, were concerning. DMO officials feared Covid-19 contracts amounted to gambling on human suffering, which is why war and terrorism markets are banned. (Similar logic doomed ex-admiral John Poindexter's Policy Analysis Market, a Bush-era plan to uncover intelligence by having security analysts bet on Middle East events.) Regulators didn't see how predicting the Grammy winners was different from betting on the Patriots to win the Super Bowl. Who, other than John Legend, would need to hedge the best R&B album winner?
Event contracts raised new questions for the DMO's product review team. Regulators could block gaming contracts that weren't in the public interest under the Commodity Exchange Act, but no one had defined gaming. It was unclear whether the CFTC had a right or an obligation to consider whether a contract was in the public interest. How was it to determine public interest? Another person familiar with the CFTC review says, "It was a mess." The agency didn't comment.
CFTC staff feared some event contracts could be cheated. Kalshi wanted to run a bee-endangerment market. The DMO pushed back, saying it saw two problems symptomatic of the asset class: traders could press government officials for information, and officials could delay adding the insects to the list to cash in.
The idea that traders might manipulate prediction markets wasn't paranoid. In 2013, academics David Rothschild and Rajiv Sethi found that an unidentified party lost $7 million buying Mitt Romney contracts on Intrade, a now-defunct, unlicensed Irish platform, in the runup to the 2012 election. The authors speculated that the trader, whom they dubbed the “Romney Whale,” may have been looking to boost morale and keep donations coming in.
Kalshi said manipulation and insider trading are risks for any market. It built a surveillance system and said it would hire a team to monitor it. "People trade on events all the time—they just use options and other instruments. This brings everything into the open, Mansour says. Kalshi didn't include election contracts, a red line for CFTC Democrats.
Lopes Lara and Mansour were ready to launch kalshi.com that summer, but the DMO blocked them. Product reviewers were frustrated by spending half their time on an exchange that represented a tiny portion of the derivatives market. Lopes Lara and Mansour pressed politically appointed commissioners during the impasse.
Tarbert, the chairman, had moved on, but Kalshi found a new supporter in Republican Brian Quintenz, a crypto-loving former hedge fund manager. He was unmoved by the DMO's concerns, arguing that speculation on Kalshi's proposed events was desirable and the agency had no legal standing to prevent it. He supported a failed bid to allow NFL futures earlier this year. Others on the commission were cautious but supportive. Given the law's ambiguity, they worried they'd be on shaky ground if Kalshi sued if they blocked a contract. Without a permanent chairman, the agency lacked leadership.
To block a contract, DMO staff needed a majority of commissioners' support, which they didn't have in all but a few cases. "We didn't have the votes," a reviewer says, paraphrasing Hamilton. By the second half of 2021, new contract requests were arriving almost daily at the DMO, and the demoralized and overrun division eventually accepted defeat and stopped fighting back. By the end of the year, three senior DMO officials had left the agency, making it easier for Kalshi to list its contracts unimpeded.
Today, Kalshi is growing. 32 employees work in a SoHo office with big windows and exposed brick. Quintenz, who left the CFTC 10 months after Kalshi was approved, is on its board. He joined because he was interested in the market's hedging and risk management opportunities.
Mid-May, the company's website had 75 markets, such as "Will Q4 GDP be negative?" Will NASA land on the moon by 2025? The exchange recently reached 2 million weekly contracts, a jump from where it started but still a small number compared to other futures exchanges. Early adopters are PredictIt and Polymarket fans. Bets on the site are currently capped at $25,000, but Kalshi hopes to increase that to $100,000 and beyond.
With the regulatory drawbridge down, Lopes Lara and Mansour must move quickly. Chicago's CME Group Inc. plans to offer index-linked event contracts. Kalshi will release a smartphone app to attract customers. After that, it hopes to partner with a big brokerage. Sequoia is a major investor in Robinhood Markets Inc. Robinhood users could have access to Kalshi so that after buying GameStop Corp. shares, they'd be prompted to bet on the Oscars or the next Fed commissioner.
Some, like Illinois Democrat Sean Casten, accuse Robinhood and its competitors of gamifying trading to encourage addiction, but Kalshi doesn't seem worried. Mansour says Kalshi's customers can't bet more than they've deposited, making debt difficult. Eventually, he may introduce leveraged bets.
Tension over event contracts recalls another CFTC episode. Brooksley Born proposed regulating the financial derivatives market in 1994. Alan Greenspan and others in the government opposed her, saying it would stifle innovation and push capital overseas. Unrestrained, derivatives grew into a trillion-dollar industry until 2008, when they sparked the financial crisis.
Today, with a midterm election looming, it seems reasonable to ask whether Kalshi plans to get involved. Elections have historically been the biggest draw in prediction markets, with 125 million shares traded on PredictIt for 2020. “We can’t discuss specifics,” Mansour says. “All I can say is, you know, we’re always working on expanding the universe of things that people can trade on.”
Any election contracts would need CFTC approval, which may be difficult with three Democratic commissioners. A Republican president would change the equation.
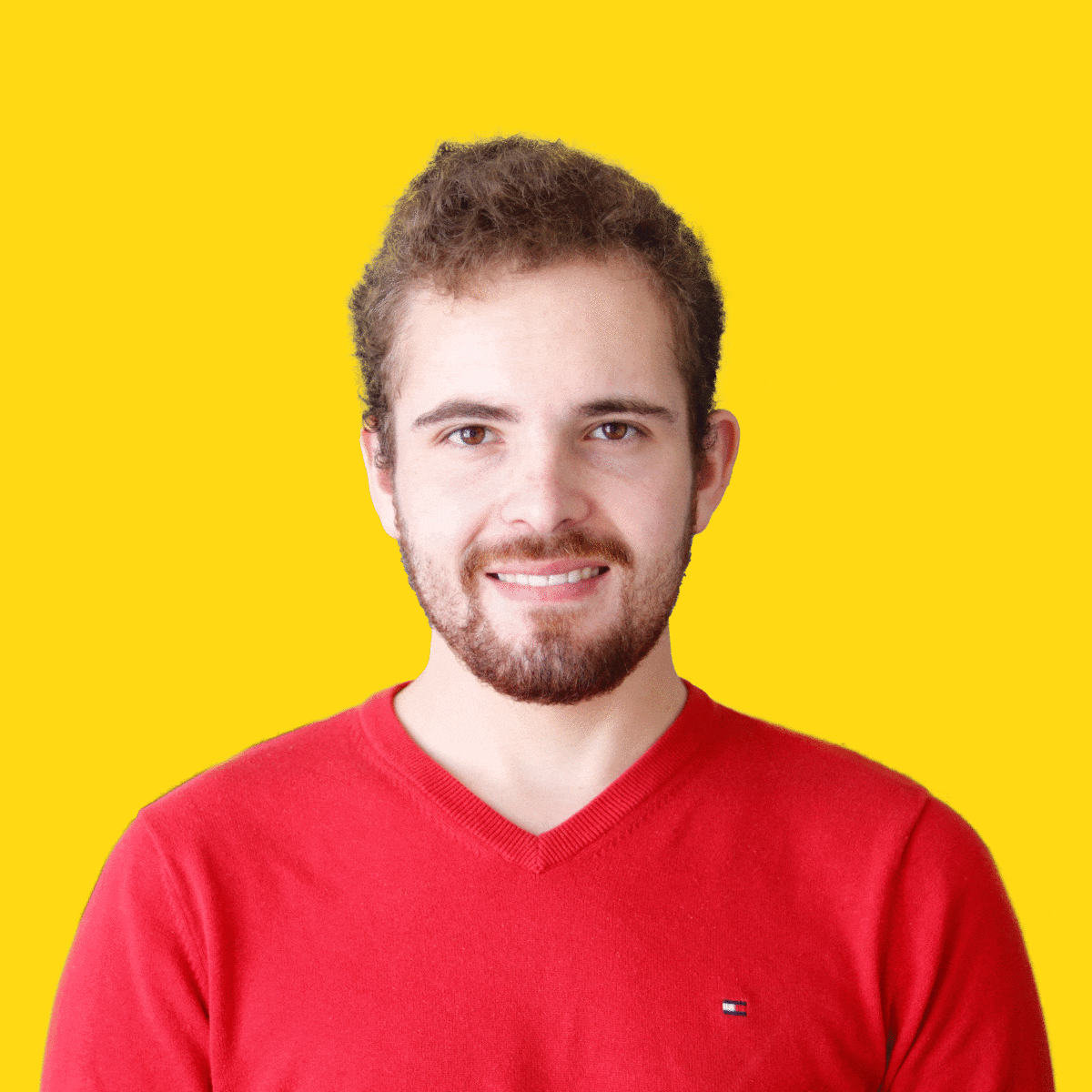
Jano le Roux
2 years ago
The Real Reason Adobe Just Paid $20 billion for Figma
Sketch or Figma?
Designers are pissed.
The beast ate the beauty.
Figma deserves $20B.
Do designers deserve Adobe?
Adobe devours new creative tools and spits them out with a slimy Adobe aftertaste.
Frame.io — $1.3B
Magento — $1.7B
Macromedia — $3.6B
Nothing compares to the risky $20B acquisition.
If they can't be beaten, buy them.
And then make them boring.
Adobe's everywhere.
Like that friend who dabbles in everything creatively, there's not enough time to master one thing.
Figma was Adobe's thigh-mounted battle axe.
a UX design instrument with a sizable free tier.
a UX design tool with a simple and quick user interface.
a tool for fluid collaboration in user experience design.
a web-based UX design tool that functions well.
a UX design tool with a singular goal of perfection.
UX design software that replaced Adobe XD.
Adobe XD could do many of Figma's things, but it didn't focus on the details. This is a major issue when working with detail-oriented professionals.
UX designers.
Design enthusiasts first used Figma. More professionals used it. Institutions taught it. Finally, major brands adopted Figma.
Adobe hated that.
Adobe dispatched a team of lawyers to resolve the Figma issue, as big companies do. Figma didn’t bite for months.
Oh no.
Figma resisted.
Figma helped designers leave Adobe. Figma couldn't replace Photoshop, but most designers used it to remove backgrounds.
Online background removal tools improved.
The Figma problem grew into a thorn, a knife, and a battle ax in Adobe's soft inner thigh.
Figma appeared to be going public. Adobe couldn’t allow that. It bought Figma for $20B during the IPO drought.
Adobe has a new issue—investors are upset.
The actual cause of investors' ire toward Adobe
Spoiler: The math just doesn’t add up.
According to Adobe's press release, Figma's annual recurring revenue (ARR) is $400M and growing rapidly.
The $20B valuation requires a 50X revenue multiple, which is unheard of.
Venture capitalists typically use:
10% to 29% growth per year: ARR multiplied by 1 to 5
30% to 99% growth per year: ARR multiplied by 6 to 10
100% to 400% growth per year: ARR multiplied by 10 to 20
Showing an investor a 50x multiple is like telling friends you saw a UFO. They'll think you're crazy.
Adobe's stock fell immediately after the acquisition because it didn't make sense to a number-cruncher.
Designers started a Tweet storm in the digital town hall where VCs and designers often meet.
Adobe acquired Workfront for $1.5 billion at the end of 2020. This purchase made sense for investors.
Many investors missed the fact that Adobe is acquiring Figma not only for its ARR but also for its brilliant collaboration tech.
Adobe could use Figmas web app technology to make more products web-based to compete with Canva.
Figma's high-profile clients could switch to Adobe's enterprise software.
However, questions arise:
Will Adobe make Figma boring?
Will Adobe tone down Figma to boost XD?
Would you ditch Adobe and Figma for Sketch?

Will Lockett
2 years ago
The World Will Change With MIT's New Battery
It's cheaper, faster charging, longer lasting, safer, and better for the environment.
Batteries are the future. Next-gen and planet-saving technology, including solar power and EVs, require batteries. As these smart technologies become more popular, we find that our batteries can't keep up. Lithium-ion batteries are expensive, slow to charge, big, fast to decay, flammable, and not environmentally friendly. MIT just created a new battery that eliminates all of these problems. So, is this the battery of the future? Or is there a catch?
When I say entirely new, I mean it. This battery employs no currently available materials. Its electrodes are constructed of aluminium and pure sulfur instead of lithium-complicated ion's metals and graphite. Its electrolyte is formed of molten chloro-aluminate salts, not an organic solution with lithium salts like lithium-ion batteries.
How does this change in materials help?
Aluminum, sulfur, and chloro-aluminate salts are abundant, easy to acquire, and cheap. This battery might be six times cheaper than a lithium-ion battery and use less hazardous mining. The world and our wallets will benefit.
But don’t go thinking this means it lacks performance.
This battery charged in under a minute in tests. At 25 degrees Celsius, the battery will charge 25 times slower than at 110 degrees Celsius. This is because the salt, which has a very low melting point, is in an ideal state at 110 degrees and can carry a charge incredibly quickly. Unlike lithium-ion, this battery self-heats when charging and discharging, therefore no external heating is needed.
Anyone who's seen a lithium-ion battery burst might be surprised. Unlike lithium-ion batteries, none of the components in this new battery can catch fire. Thus, high-temperature charging and discharging speeds pose no concern.
These batteries are long-lasting. Lithium-ion batteries don't last long, as any iPhone owner can attest. During charging, metal forms a dendrite on the electrode. This metal spike will keep growing until it reaches the other end of the battery, short-circuiting it. This is why phone batteries only last a few years and why electric car range decreases over time. This new battery's molten salt slows deposition, extending its life. This helps the environment and our wallets.
These batteries are also energy dense. Some lithium-ion batteries have 270 Wh/kg energy density (volume and mass). Aluminum-sulfur batteries could have 1392 Wh/kg, according to calculations. They'd be 5x more energy dense. Tesla's Model 3 battery would weigh 96 kg instead of 480 kg if this battery were used. This would improve the car's efficiency and handling.
These calculations were for batteries without molten salt electrolyte. Because they don't reflect the exact battery chemistry, they aren't a surefire prediction.
This battery seems great. It will take years, maybe decades, before it reaches the market and makes a difference. Right?
Nope. The project's scientists founded Avanti to develop and market this technology.
So we'll soon be driving cheap, durable, eco-friendly, lightweight, and ultra-safe EVs? Nope.
This battery must be kept hot to keep the salt molten; otherwise, it won't work and will expand and contract, causing damage. This issue could be solved by packs that can rapidly pre-heat, but that project is far off.
Rapid and constant charge-discharge cycles make these batteries ideal for solar farms, homes, and EV charging stations. The battery is constantly being charged or discharged, allowing it to self-heat and maintain an ideal temperature.
These batteries aren't as sexy as those making EVs faster, more efficient, and cheaper. Grid batteries are crucial to our net-zero transition because they allow us to use more low-carbon energy. As we move away from fossil fuels, we'll need millions of these batteries, so the fact that they're cheap, safe, long-lasting, and environmentally friendly will be huge. Who knows, maybe EVs will use this technology one day. MIT has created another world-changing technology.