More on Technology

Dmitrii Eliuseev
2 years ago
Creating Images on Your Local PC Using Stable Diffusion AI
Deep learning-based generative art is being researched. As usual, self-learning is better. Some models, like OpenAI's DALL-E 2, require registration and can only be used online, but others can be used locally, which is usually more enjoyable for curious users. I'll demonstrate the Stable Diffusion model's operation on a standard PC.
Let’s get started.
What It Does
Stable Diffusion uses numerous components:
A generative model trained to produce images is called a diffusion model. The model is incrementally improving the starting data, which is only random noise. The model has an image, and while it is being trained, the reversed process is being used to add noise to the image. Being able to reverse this procedure and create images from noise is where the true magic is (more details and samples can be found in the paper).
An internal compressed representation of a latent diffusion model, which may be altered to produce the desired images, is used (more details can be found in the paper). The capacity to fine-tune the generation process is essential because producing pictures at random is not very attractive (as we can see, for instance, in Generative Adversarial Networks).
A neural network model called CLIP (Contrastive Language-Image Pre-training) is used to translate natural language prompts into vector representations. This model, which was trained on 400,000,000 image-text pairs, enables the transformation of a text prompt into a latent space for the diffusion model in the scenario of stable diffusion (more details in that paper).
This figure shows all data flow:
The weights file size for Stable Diffusion model v1 is 4 GB and v2 is 5 GB, making the model quite huge. The v1 model was trained on 256x256 and 512x512 LAION-5B pictures on a 4,000 GPU cluster using over 150.000 NVIDIA A100 GPU hours. The open-source pre-trained model is helpful for us. And we will.
Install
Before utilizing the Python sources for Stable Diffusion v1 on GitHub, we must install Miniconda (assuming Git and Python are already installed):
wget https://repo.anaconda.com/miniconda/Miniconda3-py39_4.12.0-Linux-x86_64.sh
chmod +x Miniconda3-py39_4.12.0-Linux-x86_64.sh
./Miniconda3-py39_4.12.0-Linux-x86_64.sh
conda update -n base -c defaults conda
Install the source and prepare the environment:
git clone https://github.com/CompVis/stable-diffusion
cd stable-diffusion
conda env create -f environment.yaml
conda activate ldm
pip3 install transformers --upgrade
Download the pre-trained model weights next. HiggingFace has the newest checkpoint sd-v14.ckpt (a download is free but registration is required). Put the file in the project folder and have fun:
python3 scripts/txt2img.py --prompt "hello world" --plms --ckpt sd-v1-4.ckpt --skip_grid --n_samples 1
Almost. The installation is complete for happy users of current GPUs with 12 GB or more VRAM. RuntimeError: CUDA out of memory will occur otherwise. Two solutions exist.
Running the optimized version
Try optimizing first. After cloning the repository and enabling the environment (as previously), we can run the command:
python3 optimizedSD/optimized_txt2img.py --prompt "hello world" --ckpt sd-v1-4.ckpt --skip_grid --n_samples 1
Stable Diffusion worked on my visual card with 8 GB RAM (alas, I did not behave well enough to get NVIDIA A100 for Christmas, so 8 GB GPU is the maximum I have;).
Running Stable Diffusion without GPU
If the GPU does not have enough RAM or is not CUDA-compatible, running the code on a CPU will be 20x slower but better than nothing. This unauthorized CPU-only branch from GitHub is easiest to obtain. We may easily edit the source code to use the latest version. It's strange that a pull request for that was made six months ago and still hasn't been approved, as the changes are simple. Readers can finish in 5 minutes:
Replace if attr.device!= torch.device(cuda) with if attr.device!= torch.device(cuda) and torch.cuda.is available at line 20 of ldm/models/diffusion/ddim.py ().
Replace if attr.device!= torch.device(cuda) with if attr.device!= torch.device(cuda) and torch.cuda.is available in line 20 of ldm/models/diffusion/plms.py ().
Replace device=cuda in lines 38, 55, 83, and 142 of ldm/modules/encoders/modules.py with device=cuda if torch.cuda.is available(), otherwise cpu.
Replace model.cuda() in scripts/txt2img.py line 28 and scripts/img2img.py line 43 with if torch.cuda.is available(): model.cuda ().
Run the script again.
Testing
Test the model. Text-to-image is the first choice. Test the command line example again:
python3 scripts/txt2img.py --prompt "hello world" --plms --ckpt sd-v1-4.ckpt --skip_grid --n_samples 1
The slow generation takes 10 seconds on a GPU and 10 minutes on a CPU. Final image:
Hello world is dull and abstract. Try a brush-wielding hamster. Why? Because we can, and it's not as insane as Napoleon's cat. Another image:
Generating an image from a text prompt and another image is interesting. I made this picture in two minutes using the image editor (sorry, drawing wasn't my strong suit):
I can create an image from this drawing:
python3 scripts/img2img.py --prompt "A bird is sitting on a tree branch" --ckpt sd-v1-4.ckpt --init-img bird.png --strength 0.8
It was far better than my initial drawing:
I hope readers understand and experiment.
Stable Diffusion UI
Developers love the command line, but regular users may struggle. Stable Diffusion UI projects simplify image generation and installation. Simple usage:
Unpack the ZIP after downloading it from https://github.com/cmdr2/stable-diffusion-ui/releases. Linux and Windows are compatible with Stable Diffusion UI (sorry for Mac users, but those machines are not well-suitable for heavy machine learning tasks anyway;).
Start the script.
Done. The web browser UI makes configuring various Stable Diffusion features (upscaling, filtering, etc.) easy:
V2.1 of Stable Diffusion
I noticed the notification about releasing version 2.1 while writing this essay, and it was intriguing to test it. First, compare version 2 to version 1:
alternative text encoding. The Contrastive LanguageImage Pre-training (CLIP) deep learning model, which was trained on a significant number of text-image pairs, is used in Stable Diffusion 1. The open-source CLIP implementation used in Stable Diffusion 2 is called OpenCLIP. It is difficult to determine whether there have been any technical advancements or if legal concerns were the main focus. However, because the training datasets for the two text encoders were different, the output results from V1 and V2 will differ for the identical text prompts.
a new depth model that may be used to the output of image-to-image generation.
a revolutionary upscaling technique that can quadruple the resolution of an image.
Generally higher resolution Stable Diffusion 2 has the ability to produce both 512x512 and 768x768 pictures.
The Hugging Face website offers a free online demo of Stable Diffusion 2.1 for code testing. The process is the same as for version 1.4. Download a fresh version and activate the environment:
conda deactivate
conda env remove -n ldm # Use this if version 1 was previously installed
git clone https://github.com/Stability-AI/stablediffusion
cd stablediffusion
conda env create -f environment.yaml
conda activate ldm
Hugging Face offers a new weights ckpt file.
The Out of memory error prevented me from running this version on my 8 GB GPU. Version 2.1 fails on CPUs with the slow conv2d cpu not implemented for Half error (according to this GitHub issue, the CPU support for this algorithm and data type will not be added). The model can be modified from half to full precision (float16 instead of float32), however it doesn't make sense since v1 runs up to 10 minutes on the CPU and v2.1 should be much slower. The online demo results are visible. The same hamster painting with a brush prompt yielded this result:
It looks different from v1, but it functions and has a higher resolution.
The superresolution.py script can run the 4x Stable Diffusion upscaler locally (the x4-upscaler-ema.ckpt weights file should be in the same folder):
python3 scripts/gradio/superresolution.py configs/stable-diffusion/x4-upscaling.yaml x4-upscaler-ema.ckpt
This code allows the web browser UI to select the image to upscale:
The copy-paste strategy may explain why the upscaler needs a text prompt (and the Hugging Face code snippet does not have any text input as well). I got a GPU out of memory error again, although CUDA can be disabled like v1. However, processing an image for more than two hours is unlikely:
Stable Diffusion Limitations
When we use the model, it's fun to see what it can and can't do. Generative models produce abstract visuals but not photorealistic ones. This fundamentally limits The generative neural network was trained on text and image pairs, but humans have a lot of background knowledge about the world. The neural network model knows nothing. If someone asks me to draw a Chinese text, I can draw something that looks like Chinese but is actually gibberish because I never learnt it. Generative AI does too! Humans can learn new languages, but the Stable Diffusion AI model includes only language and image decoder brain components. For instance, the Stable Diffusion model will pull NO WAR banner-bearers like this:
V1:
V2.1:
The shot shows text, although the model never learned to read or write. The model's string tokenizer automatically converts letters to lowercase before generating the image, so typing NO WAR banner or no war banner is the same.
I can also ask the model to draw a gorgeous woman:
V1:
V2.1:
The first image is gorgeous but physically incorrect. A second one is better, although it has an Uncanny valley feel. BTW, v2 has a lifehack to add a negative prompt and define what we don't want on the image. Readers might try adding horrible anatomy to the gorgeous woman request.
If we ask for a cartoon attractive woman, the results are nice, but accuracy doesn't matter:
V1:
V2.1:
Another example: I ordered a model to sketch a mouse, which looks beautiful but has too many legs, ears, and fingers:
V1:
V2.1: improved but not perfect.
V1 produces a fun cartoon flying mouse if I want something more abstract:
I tried multiple times with V2.1 but only received this:
The image is OK, but the first version is closer to the request.
Stable Diffusion struggles to draw letters, fingers, etc. However, abstract images yield interesting outcomes. A rural landscape with a modern metropolis in the background turned out well:
V1:
V2.1:
Generative models help make paintings too (at least, abstract ones). I searched Google Image Search for modern art painting to see works by real artists, and this was the first image:
I typed "abstract oil painting of people dancing" and got this:
V1:
V2.1:
It's a different style, but I don't think the AI-generated graphics are worse than the human-drawn ones.
The AI model cannot think like humans. It thinks nothing. A stable diffusion model is a billion-parameter matrix trained on millions of text-image pairs. I input "robot is creating a picture with a pen" to create an image for this post. Humans understand requests immediately. I tried Stable Diffusion multiple times and got this:
This great artwork has a pen, robot, and sketch, however it was not asked. Maybe it was because the tokenizer deleted is and a words from a statement, but I tried other requests such robot painting picture with pen without success. It's harder to prompt a model than a person.
I hope Stable Diffusion's general effects are evident. Despite its limitations, it can produce beautiful photographs in some settings. Readers who want to use Stable Diffusion results should be warned. Source code examination demonstrates that Stable Diffusion images feature a concealed watermark (text StableDiffusionV1 and SDV2) encoded using the invisible-watermark Python package. It's not a secret, because the official Stable Diffusion repository's test watermark.py file contains a decoding snippet. The put watermark line in the txt2img.py source code can be removed if desired. I didn't discover this watermark on photographs made by the online Hugging Face demo. Maybe I did something incorrectly (but maybe they are just not using the txt2img script on their backend at all).
Conclusion
The Stable Diffusion model was fascinating. As I mentioned before, trying something yourself is always better than taking someone else's word, so I encourage readers to do the same (including this article as well;).
Is Generative AI a game-changer? My humble experience tells me:
I think that place has a lot of potential. For designers and artists, generative AI can be a truly useful and innovative tool. Unfortunately, it can also pose a threat to some of them since if users can enter a text field to obtain a picture or a website logo in a matter of clicks, why would they pay more to a different party? Is it possible right now? unquestionably not yet. Images still have a very poor quality and are erroneous in minute details. And after viewing the image of the stunning woman above, models and fashion photographers may also unwind because it is highly unlikely that AI will replace them in the upcoming years.
Today, generative AI is still in its infancy. Even 768x768 images are considered to be of a high resolution when using neural networks, which are computationally highly expensive. There isn't an AI model that can generate high-resolution photographs natively without upscaling or other methods, at least not as of the time this article was written, but it will happen eventually.
It is still a challenge to accurately represent knowledge in neural networks (information like how many legs a cat has or the year Napoleon was born). Consequently, AI models struggle to create photorealistic photos, at least where little details are important (on the other side, when I searched Google for modern art paintings, the results are often even worse;).
When compared to the carefully chosen images from official web pages or YouTube reviews, the average output quality of a Stable Diffusion generation process is actually less attractive because to its high degree of randomness. When using the same technique on their own, consumers will theoretically only view those images as 1% of the results.
Anyway, it's exciting to witness this area's advancement, especially because the project is open source. Google's Imagen and DALL-E 2 can also produce remarkable findings. It will be interesting to see how they progress.

Duane Michael
2 years ago
Don't Fall Behind: 7 Subjects You Must Understand to Keep Up with Technology
As technology develops, you should stay up to date
You don't want to fall behind, do you? This post covers 7 tech-related things you should know.
You'll learn how to operate your computer (and other electronic devices) like an expert and how to leverage the Internet and social media to create your brand and business. Read on to stay relevant in today's tech-driven environment.
You must learn how to code.
Future-language is coding. It's how we and computers talk. Learn coding to keep ahead.
Try Codecademy or Code School. There are also numerous free courses like Coursera or Udacity, but they take a long time and aren't necessarily self-paced, so it can be challenging to find the time.
Artificial intelligence (AI) will transform all jobs.
Our skillsets must adapt with technology. AI is a must-know topic. AI will revolutionize every employment due to advances in machine learning.
Here are seven AI subjects you must know.
What is artificial intelligence?
How does artificial intelligence work?
What are some examples of AI applications?
How can I use artificial intelligence in my day-to-day life?
What jobs have a high chance of being replaced by artificial intelligence and how can I prepare for this?
Can machines replace humans? What would happen if they did?
How can we manage the social impact of artificial intelligence and automation on human society and individual people?
Blockchain Is Changing the Future
Few of us know how Bitcoin and blockchain technology function or what impact they will have on our lives. Blockchain offers safe, transparent, tamper-proof transactions.
It may alter everything from business to voting. Seven must-know blockchain topics:
Describe blockchain.
How does the blockchain function?
What advantages does blockchain offer?
What possible uses for blockchain are there?
What are the dangers of blockchain technology?
What are my options for using blockchain technology?
What does blockchain technology's future hold?
Cryptocurrencies are here to stay
Cryptocurrencies employ cryptography to safeguard transactions and manage unit creation. Decentralized cryptocurrencies aren't controlled by governments or financial institutions.
Bitcoin, the first cryptocurrency, was launched in 2009. Cryptocurrencies can be bought and sold on decentralized exchanges.
Bitcoin is here to stay.
Bitcoin isn't a fad, despite what some say. Since 2009, Bitcoin's popularity has grown. Bitcoin is worth learning about now. Since 2009, Bitcoin has developed steadily.
With other cryptocurrencies emerging, many people are wondering if Bitcoin still has a bright future. Curiosity is natural. Millions of individuals hope their Bitcoin investments will pay off since they're popular now.
Thankfully, they will. Bitcoin is still running strong a decade after its birth. Here's why.
The Internet of Things (IoT) is no longer just a trendy term.
IoT consists of internet-connected physical items. These items can share data. IoT is young but developing fast.
20 billion IoT-connected devices are expected by 2023. So much data! All IT teams must keep up with quickly expanding technologies. Four must-know IoT topics:
Recognize the fundamentals: Priorities first! Before diving into more technical lingo, you should have a fundamental understanding of what an IoT system is. Before exploring how something works, it's crucial to understand what you're working with.
Recognize Security: Security does not stand still, even as technology advances at a dizzying pace. As IT professionals, it is our duty to be aware of the ways in which our systems are susceptible to intrusion and to ensure that the necessary precautions are taken to protect them.
Be able to discuss cloud computing: The cloud has seen various modifications over the past several years once again. The use of cloud computing is also continually changing. Knowing what kind of cloud computing your firm or clients utilize will enable you to make the appropriate recommendations.
Bring Your Own Device (BYOD)/Mobile Device Management (MDM) is a topic worth discussing (MDM). The ability of BYOD and MDM rules to lower expenses while boosting productivity among employees who use these services responsibly is a major factor in their continued growth in popularity.
IoT Security is key
As more gadgets connect, they must be secure. IoT security includes securing devices and encrypting data. Seven IoT security must-knows:
fundamental security ideas
Authorization and identification
Cryptography
electronic certificates
electronic signatures
Private key encryption
Public key encryption
Final Thoughts
With so much going on in the globe, it can be hard to stay up with technology. We've produced a list of seven tech must-knows.

Asha Barbaschow
3 years ago
Apple WWDC 2022 Announcements
WWDC 2022 began early Tuesday morning. WWDC brought a ton of new features (which went for just shy of two hours).
With so many announcements, we thought we'd compile them. And now...
WWDC?
WWDC is Apple's developer conference. This includes iOS, macOS, watchOS, and iPadOS (all of its iPads). It's where Apple announces new features for developers to use. It's also where Apple previews new software.
Virtual WWDC runs June 6-10. You can rewatch the stream on Apple's website.
WWDC 2022 news:
Completely everything. Really. iOS 16 first.
iOS 16.
iOS 16 is a major iPhone update. iOS 16 adds the ability to customize the Lock Screen's color/theme. And widgets. It also organizes notifications and pairs Lock Screen with Focus themes. Edit or recall recently sent messages, recover recently deleted messages, and mark conversations as unread. Apple gives us yet another reason to stay in its walled garden with iMessage.
New iOS includes family sharing. Parents can set up a child's account with parental controls to restrict apps, movies, books, and music. iOS 16 lets large families and friend pods share iCloud photos. Up to six people can contribute photos to a separate iCloud library.
Live Text is getting creepier. Users can interact with text in any video frame. Touch and hold an image's subject to remove it from its background and place it in apps like messages. Dictation offers a new on-device voice-and-touch experience. Siri can run app shortcuts without setup in iOS 16. Apple also unveiled a new iOS 16 feature to help people break up with abusive partners who track their locations or read their messages. Safety Check.
Apple Pay Later allows iPhone users to buy products and pay for them later. iOS 16 pushes Mail. Users can schedule emails and cancel delivery before it reaches a recipient's inbox (be quick!). Mail now detects if you forgot an attachment, as Gmail has for years. iOS 16's Maps app gets "Multi-Stop Routing," .
Apple News also gets an iOS 16 update. Apple News adds My Sports. With iOS 16, the Apple Watch's Fitness app is also coming to iOS and the iPhone, using motion-sensing tech to track metrics and performance (as long as an athlete is wearing or carrying the device on their person).
iOS 16 includes accessibility updates like Door Detection.
watchOS9
Many of Apple's software updates are designed to take advantage of the larger screens in recent models, but they also improve health and fitness tracking.
The most obvious reason to upgrade watchOS every year is to get new watch faces from Apple. WatchOS 9 will add four new faces.
Runners' workout metrics improve.
Apple quickly realized that fitness tracking would be the Apple Watch's main feature, even though it's been the killer app for wearables since their debut. For watchOS 9, the Apple Watch will use its accelerometer and gyroscope to track a runner's form, stride length, and ground contact time. It also introduces the ability to specify heart rate zones, distance, and time intervals, with vibrating haptic feedback and voice alerts.
The Apple Watch's Fitness app is coming to iOS and the iPhone, using the smartphone's motion-sensing tech to track metrics and performance (as long as an athlete is wearing or carrying the device on their person).
We'll get sleep tracking, medication reminders, and drug interaction alerts. Your watch can create calendar events. A new Week view shows what meetings or responsibilities stand between you and the weekend.
iPadOS16
WWDC 2022 introduced iPad updates. iPadOS 16 is similar to iOS for the iPhone, but has features for larger screens and tablet accessories. The software update gives it many iPhone-like features.
iPadOS 16's Home app, like iOS 16, will have a new design language. iPad users who want to blame it on the rain finally have a Weather app. iPadOS 16 will have iCloud's Shared Photo Library, Live Text and Visual Look Up upgrades, and FaceTime Handoff, so you can switch between devices during a call.
Apple highlighted iPadOS 16's multitasking at WWDC 2022. iPad's Stage Manager sounds like a community theater app. It's a powerful multitasking tool for tablets and brings them closer to emulating laptops. Apple's iPadOS 16 supports multi-user collaboration. You can share content from Files, Keynote, Numbers, Pages, Notes, Reminders, Safari, and other third-party apps in Apple Messages.
M2-chip
WWDC 2022 revealed Apple's M2 chip. Apple has started the next generation of Apple Silicon for the Mac with M2. Apple says this device improves M1's performance.
M2's second-generation 5nm chip has 25% more transistors than M1's. 100GB/s memory bandwidth (50 per cent more than M1). M2 has 24GB of unified memory, up from 16GB but less than some ultraportable PCs' 32GB. The M2 chip has 10% better multi-core CPU performance than the M2, and it's nearly twice as fast as the latest 10-core PC laptop chip at the same power level (CPU performance is 18 per cent greater than M1).
New MacBooks
Apple introduced the M2-powered MacBook Air. Apple's entry-level laptop has a larger display, a new processor, new colors, and a notch.
M2 also powers the 13-inch MacBook Pro. The 13-inch MacBook Pro has 24GB of unified memory and 50% more memory bandwidth. New MacBook Pro batteries last 20 hours. As I type on the 2021 MacBook Pro, I can only imagine how much power the M2 will add.
macOS 13.0 (or, macOS Ventura)
macOS Ventura will take full advantage of M2 with new features like Stage Manager and Continuity Camera and Handoff for FaceTime. Safari, Mail, Messages, Spotlight, and more get updates in macOS Ventura.
Apple hasn't run out of California landmarks to name its OS after yet. macOS 13 will be called Ventura when it's released in a few months, but it's more than a name change and new wallpapers.
Stage Manager organizes windows
Stage Manager is a new macOS tool that organizes open windows and applications so they're still visible while focusing on a specific task. The main app sits in the middle of the desktop, while other apps and documents are organized and piled up to the side.
Improved Searching
Spotlight is one of macOS's least appreciated features, but with Ventura, it's becoming even more useful. Live Text lets you extract text from Spotlight results without leaving the window, including images from the photo library and the web.
Mail lets you schedule or unsend emails.
We've all sent an email we regret, whether it contained regrettable words or was sent at the wrong time. In macOS Ventura, Mail users can cancel or reschedule a message after sending it. Mail will now intelligently determine if a person was forgotten from a CC list or if a promised attachment wasn't included. Procrastinators can set a reminder to read a message later.
Safari adds tab sharing and password passkeys
Apple is updating Safari to make it more user-friendly... mostly. Users can share a group of tabs with friends or family, a useful feature when researching a topic with too many tabs. Passkeys will replace passwords in Safari's next version. Instead of entering random gibberish when creating a new account, macOS users can use TouchID to create an on-device passkey. Using an iPhone's camera and a QR system, Passkey syncs and works across all Apple devices and Windows computers.
Continuity adds Facetime device switching and iPhone webcam.
With macOS Ventura, iPhone users can transfer a FaceTime call from their phone to their desktop or laptop using Handoff, or vice versa if they started a call at their desk and need to continue it elsewhere. Apple finally admits its laptop and monitor webcams aren't the best. Continuity makes the iPhone a webcam. Apple demonstrated a feature where the wide-angle lens could provide a live stream of the desk below, while the standard zoom lens could focus on the speaker's face. New iPhone laptop mounts are coming.
System Preferences
System Preferences is Now System Settings and Looks Like iOS
Ventura's System Preferences has been renamed System Settings and is much more similar in appearance to iOS and iPadOS. As the iPhone and iPad are gateway devices into Apple's hardware ecosystem, new Mac users should find it easier to adjust.
This post is a summary. Read full article here
You might also like
Guillaume Dumortier
2 years ago
Mastering the Art of Rhetoric: A Guide to Rhetorical Devices in Successful Headlines and Titles
Unleash the power of persuasion and captivate your audience with compelling headlines.
As the old adage goes, "You never get a second chance to make a first impression."
In the world of content creation and social ads, headlines and titles play a critical role in making that first impression.
A well-crafted headline can make the difference between an article being read or ignored, a video being clicked on or bypassed, or a product being purchased or passed over.
To make an impact with your headlines, mastering the art of rhetoric is essential. In this post, we'll explore various rhetorical devices and techniques that can help you create headlines that captivate your audience and drive engagement.
tl;dr : Headline Magician will help you craft the ultimate headline titles powered by rhetoric devices
Example with a high-end luxury organic zero-waste skincare brand
✍️ The Power of Alliteration
Alliteration is the repetition of the same consonant sound at the beginning of words in close proximity. This rhetorical device lends itself well to headlines, as it creates a memorable, rhythmic quality that can catch a reader's attention.
By using alliteration, you can make your headlines more engaging and easier to remember.
Examples:
"Crafting Compelling Content: A Comprehensive Course"
"Mastering the Art of Memorable Marketing"
🔁 The Appeal of Anaphora
Anaphora is the repetition of a word or phrase at the beginning of successive clauses. This rhetorical device emphasizes a particular idea or theme, making it more memorable and persuasive.
In headlines, anaphora can be used to create a sense of unity and coherence, which can draw readers in and pique their interest.
Examples:
"Create, Curate, Captivate: Your Guide to Social Media Success"
"Innovation, Inspiration, and Insight: The Future of AI"
🔄 The Intrigue of Inversion
Inversion is a rhetorical device where the normal order of words is reversed, often to create an emphasis or achieve a specific effect.
In headlines, inversion can generate curiosity and surprise, compelling readers to explore further.
Examples:
"Beneath the Surface: A Deep Dive into Ocean Conservation"
"Beyond the Stars: The Quest for Extraterrestrial Life"
⚖️ The Persuasive Power of Parallelism
Parallelism is a rhetorical device that involves using similar grammatical structures or patterns to create a sense of balance and symmetry.
In headlines, parallelism can make your message more memorable and impactful, as it creates a pleasing rhythm and flow that can resonate with readers.
Examples:
"Eat Well, Live Well, Be Well: The Ultimate Guide to Wellness"
"Learn, Lead, and Launch: A Blueprint for Entrepreneurial Success"
⏭️ The Emphasis of Ellipsis
Ellipsis is the omission of words, typically indicated by three periods (...), which suggests that there is more to the story.
In headlines, ellipses can create a sense of mystery and intrigue, enticing readers to click and discover what lies behind the headline.
Examples:
"The Secret to Success... Revealed"
"Unlocking the Power of Your Mind... A Step-by-Step Guide"
🎭 The Drama of Hyperbole
Hyperbole is a rhetorical device that involves exaggeration for emphasis or effect.
In headlines, hyperbole can grab the reader's attention by making bold, provocative claims that stand out from the competition. Be cautious with hyperbole, however, as overuse or excessive exaggeration can damage your credibility.
Examples:
"The Ultimate Guide to Mastering Any Skill in Record Time"
"Discover the Revolutionary Technique That Will Transform Your Life"
❓The Curiosity of Questions
Posing questions in your headlines can be an effective way to pique the reader's curiosity and encourage engagement.
Questions compel the reader to seek answers, making them more likely to click on your content. Additionally, questions can create a sense of connection between the content creator and the audience, fostering a sense of dialogue and discussion.
Examples:
"Are You Making These Common Mistakes in Your Marketing Strategy?"
"What's the Secret to Unlocking Your Creative Potential?"
💥 The Impact of Imperatives
Imperatives are commands or instructions that urge the reader to take action. By using imperatives in your headlines, you can create a sense of urgency and importance, making your content more compelling and actionable.
Examples:
"Master Your Time Management Skills Today"
"Transform Your Business with These Innovative Strategies"
💢 The Emotion of Exclamations
Exclamations are powerful rhetorical devices that can evoke strong emotions and convey a sense of excitement or urgency.
Including exclamations in your headlines can make them more attention-grabbing and shareable, increasing the chances of your content being read and circulated.
Examples:
"Unlock Your True Potential: Find Your Passion and Thrive!"
"Experience the Adventure of a Lifetime: Travel the World on a Budget!"
🎀 The Effectiveness of Euphemisms
Euphemisms are polite or indirect expressions used in place of harsher, more direct language.
In headlines, euphemisms can make your message more appealing and relatable, helping to soften potentially controversial or sensitive topics.
Examples:
"Navigating the Challenges of Modern Parenting"
"Redefining Success in a Fast-Paced World"
⚡Antithesis: The Power of Opposites
Antithesis involves placing two opposite words side-by-side, emphasizing their contrasts. This device can create a sense of tension and intrigue in headlines.
Examples:
"Once a day. Every day"
"Soft on skin. Kill germs"
"Mega power. Mini size."
To utilize antithesis, identify two opposing concepts related to your content and present them in a balanced manner.
🎨 Scesis Onomaton: The Art of Verbless Copy
Scesis onomaton is a rhetorical device that involves writing verbless copy, which quickens the pace and adds emphasis.
Example:
"7 days. 7 dollars. Full access."
To use scesis onomaton, remove verbs and focus on the essential elements of your headline.
🌟 Polyptoton: The Charm of Shared Roots
Polyptoton is the repeated use of words that share the same root, bewitching words into memorable phrases.
Examples:
"Real bread isn't made in factories. It's baked in bakeries"
"Lose your knack for losing things."
To employ polyptoton, identify words with shared roots that are relevant to your content.
✨ Asyndeton: The Elegance of Omission
Asyndeton involves the intentional omission of conjunctions, adding crispness, conviction, and elegance to your headlines.
Examples:
"You, Me, Sushi?"
"All the latte art, none of the environmental impact."
To use asyndeton, eliminate conjunctions and focus on the core message of your headline.
🔮 Tricolon: The Magic of Threes
Tricolon is a rhetorical device that uses the power of three, creating memorable and impactful headlines.
Examples:
"Show it, say it, send it"
"Eat Well, Live Well, Be Well."
To use tricolon, craft a headline with three key elements that emphasize your content's main message.
🔔 Epistrophe: The Chime of Repetition
Epistrophe involves the repetition of words or phrases at the end of successive clauses, adding a chime to your headlines.
Examples:
"Catch it. Bin it. Kill it."
"Joint friendly. Climate friendly. Family friendly."
To employ epistrophe, repeat a key phrase or word at the end of each clause.
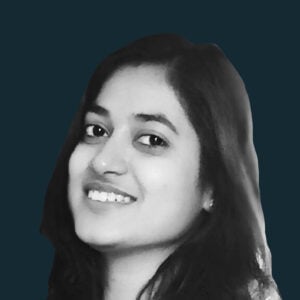
Yogita Khatri
3 years ago
Moonbirds NFT sells for $1 million in first week
On Saturday, Moonbird #2642, one of the collection's rarest NFTs, sold for a record 350 ETH (over $1 million) on OpenSea.
The Sandbox, a blockchain-based gaming company based in Hong Kong, bought the piece. The seller, "oscuranft" on OpenSea, made around $600,000 after buying the NFT for 100 ETH a week ago.
Owl avatars
Moonbirds is a 10,000 owl NFT collection. It is one of the quickest collections to achieve bluechip status. Proof, a media startup founded by renowned VC Kevin Rose, launched Moonbirds on April 16.
Rose is currently a partner at True Ventures, a technology-focused VC firm. He was a Google Ventures general partner and has 1.5 million Twitter followers.
Rose has an NFT podcast on Proof. It follows Proof Collective, a group of 1,000 NFT collectors and artists, including Beeple, who hold a Proof Collective NFT and receive special benefits.
These include early access to the Proof podcast and in-person events.
According to the Moonbirds website, they are "the official Proof PFP" (picture for proof).
Moonbirds NFTs sold nearly $360 million in just over a week, according to The Block Research and Dune Analytics. Its top ten sales range from $397,000 to $1 million.
In the current market, Moonbirds are worth 33.3 ETH. Each NFT is 2.5 ETH. Holders have gained over 12 times in just over a week.
Why was it so popular?
The Block Research's NFT analyst, Thomas Bialek, attributes Moonbirds' rapid rise to Rose's backing, the success of his previous Proof Collective project, and collectors' preference for proven NFT projects.
Proof Collective NFT holders have made huge gains. These NFTs were sold in a Dutch auction last December for 5 ETH each. According to OpenSea, the current floor price is 109 ETH.
According to The Block Research, citing Dune Analytics, Proof Collective NFTs have sold over $39 million to date.
Rose has bigger plans for Moonbirds. Moonbirds is introducing "nesting," a non-custodial way for holders to stake NFTs and earn rewards.
Holders of NFTs can earn different levels of status based on how long they keep their NFTs locked up.
"As you achieve different nest status levels, we can offer you different benefits," he said. "We'll have in-person meetups and events, as well as some crazy airdrops planned."
Rose went on to say that Proof is just the start of "a multi-decade journey to build a new media company."

Sad NoCoiner
2 years ago
Two Key Money Principles You Should Understand But Were Never Taught
Prudence is advised. Be debt-free. Be frugal. Spend less.
This advice sounds nice, but it rarely works.
Most people never learn these two money rules. Both approaches will impact how you see personal finance.
It may safeguard you from inflation or the inability to preserve money.
Let’s dive in.
#1: Making long-term debt your ally
High-interest debt hurts consumers. Many credit cards carry 25% yearly interest (or more), so always pay on time. Otherwise, you’re losing money.
Some low-interest debt is good. Especially when buying an appreciating asset with borrowed money.
Inflation helps you.
If you borrow $800,000 at 3% interest and invest it at 7%, you'll make $32,000 (4%).
As money loses value, fixed payments get cheaper. Your assets' value and cash flow rise.
The never-in-debt crowd doesn't know this. They lose money paying off mortgages and low-interest loans early when they could have bought assets instead.
#2: How To Buy Or Build Assets To Make Inflation Irrelevant
Dozens of studies demonstrate actual wage growth is static; $2.50 in 1964 was equivalent to $22.65 now.
These reports never give solutions unless they're selling gold.
But there is one.
Assets beat inflation.
$100 invested into the S&P 500 would have an inflation-adjusted return of 17,739.30%.
Likewise, you can build assets from nothing. Doing is easy and quick. The returns can boost your income by 10% or more.
The people who obsess over inflation inadvertently make the problem worse for themselves. They wait for The Big Crash to buy assets. Or they moan about debt clocks and spending bills instead of seeking a solution.
Conclusion
Being ultra-prudent is like playing golf with a putter to avoid hitting the ball into the water. Sure, you might not slice a drive into the pond. But, you aren’t going to play well either. Or have very much fun.
Money has rules.
Avoiding debt or investment risks will limit your rewards. Long-term, being too cautious hurts your finances.
Disclaimer: This article is for entertainment purposes only. It is not financial advice, always do your own research.