More on Entrepreneurship/Creators

Jenn Leach
1 year ago
What TikTok Paid Me in 2021 with 100,000 Followers
I thought it would be interesting to share how much TikTok paid me in 2021.
Onward!
Oh, you get paid by TikTok?
Yes.
They compensate thousands of creators. My Tik Tok account
I launched my account in March 2020 and generally post about money, finance, and side hustles.
TikTok creators are paid in several ways.
Fund for TikTok creators
Sponsorships (aka brand deals)
Affiliate promotion
My own creations
Only one, the TikTok Creator Fund, pays me.
The TikTok Creator Fund: What Is It?
TikTok's initiative pays creators.
YouTube's Shorts Fund, Snapchat Spotlight, and other platforms have similar programs.
Creator Fund doesn't pay everyone. Some prerequisites are:
age requirement of at least 18 years
In the past 30 days, there must have been 100,000 views.
a minimum of 10,000 followers
If you qualify, you can apply using your TikTok account, and once accepted, your videos can earn money.
My earnings from the TikTok Creator Fund
Since 2020, I've made $273.65. My 2021 payment is $77.36.
Yikes!
I made between $4.91 to around $13 payout each time I got paid.
TikTok reportedly pays 3 to 5 cents per thousand views.
To live off the Creator Fund, you'd need billions of monthly views.
Top personal finance creator Sara Finance has millions (if not billions) of views and over 700,000 followers yet only received $3,000 from the TikTok Creator Fund.
Goals for 2022
TikTok pays me in different ways, as listed above.
My largest TikTok account isn't my only one.
In 2022, I'll revamp my channel.
It's been a tumultuous year on TikTok for my account, from getting shadow-banned to being banned from the Creator Fund to being accepted back (not at my wish).
What I've experienced isn't rare. I've read about other creators' experiences.
So, some quick goals for this account…
200,000 fans by the year 2023
Consistent monthly income of $5,000
two brand deals each month
For now, that's all.

MAJESTY AliNICOLE WOW!
1 year ago
YouTube's faceless videos are growing in popularity, but this is nothing new.
I've always bucked social media norms. YouTube doesn't compare. Traditional video made me zig when everyone zagged. Audio, picture personality animation, thought movies, and slide show videos are most popular and profitable.
YouTube's business is shifting. While most video experts swear by the idea that YouTube success is all about making personal and professional Face-Share-Videos, those who use YouTube for business know things are different.
In this article, I will share concepts from my mini master class Figures to Followers: Prioritizing Purposeful Profits Over Popularity on YouTube to Create the Win-Win for You, Your Audience & More and my forthcoming publication The WOWTUBE-PRENEUR FACTOR EVOLUTION: The Basics of Powerfully & Profitably Positioning Yourself as a Video Communications Authority to Broadcast Your WOW Effect as a Video Entrepreneur.
I've researched the psychology, anthropology, and anatomy of significant social media platforms as an entrepreneur and social media marketing expert. While building my YouTube empire, I've paid particular attention to what works for short, mid, and long-term success, whether it's a niche-focused, lifestyle, or multi-interest channel.
Most new, semi-new, and seasoned YouTubers feel vlog-style or live-on-camera videos are popular. Faceless, animated, music-text-based, and slideshow videos do well for businesses.
Buyer-consumer vs. content-consumer thinking is totally different when absorbing content. Profitability and popularity are closely related, however most people become popular with traditional means but not profitable.
In my experience, Faceless videos are more profitable, although it depends on the channel's style. Several professionals are now teaching in their courses that non-traditional films are making the difference in their business success and popularity.
Face-Share-Personal-Touch videos make audiences feel like they know the personality, but they're not profitable.
Most spend hours creating articles, videos, and thumbnails to seem good. That's how most YouTubers gained their success in the past, but not anymore.
Looking the part and performing a typical role in videos doesn't convert well, especially for newbie channels.
Working with video marketers and YouTubers for years, I've noticed that most struggle to be consistent with content publishing since they exclusively use formats that need extensive development. Camera and green screen set ups, shooting/filming, and editing for post productions require their time, making it less appealing to post consistently, especially if they're doing all the work themselves.
Because they won't make simple format videos or audio videos with an overlay image, they overcomplicate the procedure (even with YouTube Shorts), and they leave their channels for weeks or months. Again, they believe YouTube only allows specific types of videos. Even though this procedure isn't working, they plan to keep at it.
A successful YouTube channel needs multiple video formats to suit viewer needs, I teach. Face-Share-Personal Touch and Faceless videos are both useful.
How people engage with YouTube content has changed over the years, and the average customer is no longer interested in an all-video channel.
Face-Share-Personal-Touch videos are great
Google Live
Online training
Giving listeners a different way to access your podcast that is being broadcast on sites like Anchor, BlogTalkRadio, Spreaker, Google, Apple Store, and others Many people enjoy using a video camera to record themselves while performing the internet radio, Facebook, or Instagram Live versions of their podcasts.
Video Blog Updates
even more
Faceless videos are popular for business and benefit both entrepreneurs and audiences.
For the business owner/entrepreneur…
Less production time results in time dollar savings.
enables the business owner to demonstrate the diversity of content development
For the Audience…
The channel offers a variety of appealing content options.
The same format is not monotonous or overly repetitive for the viewers.
Below are a couple videos from YouTube guru Make Money Matt's channel, which has over 347K subscribers.
Enjoy
24 Best Niches to Make Money on YouTube Without Showing Your Face
Make Money on YouTube Without Making Videos (Free Course)
In conclusion, you have everything it takes to build your own YouTube brand and empire. Learn the rules, then adapt them to succeed.
Please reread this and the other suggested articles for optimal benefit.
I hope this helped. How has this article helped you? Follow me for more articles like this and more multi-mission expressions.
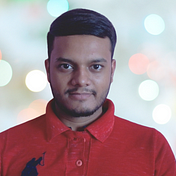
Sanjay Priyadarshi
1 year ago
Meet a Programmer Who Turned Down Microsoft's $10,000,000,000 Acquisition Offer
Failures inspire young developers
Jason citron created many products.
These products flopped.
Microsoft offered $10 billion for one of these products.
He rejected the offer since he was so confident in his success.
Let’s find out how he built a product that is currently valued at $15 billion.
Early in his youth, Jason began learning to code.
Jason's father taught him programming and IT.
His father wanted to help him earn money when he needed it.
Jason created video games and websites in high school.
Jason realized early on that his IT and programming skills could make him money.
Jason's parents misjudged his aptitude for programming.
Jason frequented online programming communities.
He looked for web developers. He created websites for those people.
His parents suspected Jason sold drugs online. When he said he used programming to make money, they were shocked.
They helped him set up a PayPal account.
Florida higher education to study video game creation
Jason never attended an expensive university.
He studied game design in Florida.
“Higher Education is an interesting part of society… When I work with people, the school they went to never comes up… only thing that matters is what can you do…At the end of the day, the beauty of silicon valley is that if you have a great idea and you can bring it to the life, you can convince a total stranger to give you money and join your project… This notion that you have to go to a great school didn’t end up being a thing for me.”
Jason's life was altered by Steve Jobs' keynote address.
After graduating, Jason joined an incubator.
Jason created a video-dating site first.
Bad idea.
Nobody wanted to use it when it was released, so they shut it down.
He made a multiplayer game.
It was released on Bebo. 10,000 people played it.
When Steve Jobs unveiled the Apple app store, he stopped playing.
The introduction of the app store resembled that of a new gaming console.
Jason's life altered after Steve Jobs' 2008 address.
“Whenever a new video game console is launched, that’s the opportunity for a new video game studio to get started, it’s because there aren’t too many games available…When a new PlayStation comes out, since it’s a new system, there’s only a handful of titles available… If you can be a launch title you can get a lot of distribution.”
Apple's app store provided a chance to start a video game company.
They released an app after 5 months of work.
Aurora Feint is the game.
Jason believed 1000 players in a week would be wonderful. A thousand players joined in the first hour.
Over time, Aurora Feints' game didn't gain traction. They don't make enough money to keep playing.
They could only make enough for one month.
Instead of buying video games, buy technology
Jason saw that they established a leaderboard, chat rooms, and multiplayer capabilities and believed other developers would want to use these.
They opted to sell the prior game's technology.
OpenFeint.
Assisting other game developers
They had no money in the bank to create everything needed to make the technology user-friendly.
Jason and Daniel designed a website saying:
“If you’re making a video game and want to have a drop in multiplayer support, you can use our system”
TechCrunch covered their website launch, and they gained a few hundred mailing list subscribers.
They raised seed funding with the mailing list.
Nearly all iPhone game developers started adopting the Open Feint logo.
“It was pretty wild… It was really like a whole social platform for people to play with their friends.”
What kind of a business model was it?
OpenFeint originally planned to make the software free for all games. As the game gained popularity, they demanded payment.
They later concluded it wasn't a good business concept.
It became free eventually.
Acquired for $104 million
Open Feint's users and employees grew tremendously.
GREE bought OpenFeint for $104 million in April 2011.
GREE initially committed to helping Jason and his team build a fantastic company.
Three or four months after the acquisition, Jason recognized they had a different vision.
He quit.
Jason's Original Vision for the iPad
Jason focused on distribution in 2012 to help businesses stand out.
The iPad market and user base were growing tremendously.
Jason said the iPad may replace mobile gadgets.
iPad gamers behaved differently than mobile gamers.
People sat longer and experienced more using an iPad.
“The idea I had was what if we built a gaming business that was more like traditional video games but played on tablets as opposed to some kind of mobile game that I’ve been doing before.”
Unexpected insight after researching the video game industry
Jason learned from studying the gaming industry that long-standing companies had advantages beyond a single release.
Previously, long-standing video game firms had their own distribution system. This distribution strategy could buffer time between successful titles.
Sony, Microsoft, and Valve all have gaming consoles and online stores.
So he built a distribution system.
He created a group chat app for gamers.
He envisioned a team-based multiplayer game with text and voice interaction.
His objective was to develop a communication network, release more games, and start a game distribution business.
Remaking the video game League of Legends
Jason and his crew reimagined a League of Legends game mode for 12-inch glass.
They adapted the game for tablets.
League of Legends was PC-only.
So they rebuilt it.
They overhauled the game and included native mobile experiences to stand out.
Hammer and Chisel was the company's name.
18 people worked on the game.
The game was funded. The game took 2.5 years to make.
Was the game a success?
July 2014 marked the game's release. The team's hopes were dashed.
Critics initially praised the game.
Initial installation was widespread.
The game failed.
As time passed, the team realized iPad gaming wouldn't increase much and mobile would win.
Jason was given a fresh idea by Stan Vishnevskiy.
Stan Vishnevskiy was a corporate engineer.
He told Jason about his plan to design a communication app without a game.
This concept seeded modern strife.
“The insight that he really had was to put a couple of dots together… we’re seeing our customers communicating around our own game with all these different apps and also ourselves when we’re playing on PC… We should solve that problem directly rather than needing to build a new game…we should start making it on PC.”
So began Discord.
Online socializing with pals was the newest trend.
Jason grew up playing video games with his friends.
He never played outside.
Jason had many great moments playing video games with his closest buddy, wife, and brother.
Discord was about providing a location for you and your group to speak and hang out.
Like a private cafe, bedroom, or living room.
Discord was developed for you and your friends on computers and phones.
You can quickly call your buddies during a game to conduct a conference call. Put the call on speaker and talk while playing.
Discord wanted to give every player a unique experience. Because coordinating across apps was a headache.
The entire team started concentrating on Discord.
Jason decided Hammer and Chisel would focus on their chat app.
Jason didn't want to make a video game.
How Discord attracted the appropriate attention
During the first five months, the entire team worked on the game and got feedback from friends.
This ensures product improvement. As a result, some teammates' buddies started utilizing Discord.
The team knew it would become something, but the result was buggy. App occasionally crashed.
Jason persuaded a gamer friend to write on Reddit about the software.
New people would find Discord. Why not?
Reddit users discovered Discord and 50 started using it frequently.
Discord was launched.
Rejecting the $10 billion acquisition proposal
Discord has increased in recent years.
It sends billions of messages.
Discord's users aren't tracked. They're privacy-focused.
Purchase offer
Covid boosted Discord's user base.
Weekly, billions of messages were transmitted.
Microsoft offered $10 billion for Discord in 2021.
Jason sold Open Feint for $104m in 2011.
This time, he believed in the product so much that he rejected Microsoft's offer.
“I was talking to some people in the team about which way we could go… The good thing was that most of the team wanted to continue building.”
Last time, Discord was valued at $15 billion.
Discord raised money on March 12, 2022.
The $15 billion corporation raised $500 million in 2021.
You might also like
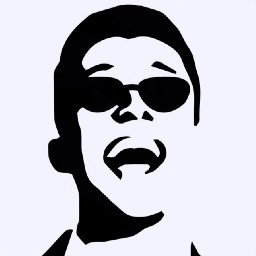
Joseph Mavericks
1 year ago
Apples Top 100 Meeting: Steve Jobs's Secret Agenda's Lessons
Jobs' secret emails became public due to a litigation with Samsung.
Steve Jobs sent Phil Schiller an email at the end of 2010. Top 100 A was the codename for Apple's annual Top 100 executive meetings. The 2011 one was scheduled.
Everything about this gathering is secret, even attendance. The location is hidden, and attendees can't even drive themselves. Instead, buses transport them to a 2-3 day retreat.
Due to a litigation with Samsung, this Top 100 meeting's agenda was made public in 2014. This was a critical milestone in Apple's history, not a Top 100 meeting. Apple had many obstacles in the 2010s to remain a technological leader. Apple made more money with non-PC goods than with its best-selling Macintosh series. This was the last Top 100 gathering Steve Jobs would attend before passing, and he wanted to make sure his messages carried on before handing over his firm to Tim Cook.
In this post, we'll discuss lessons from Jobs' meeting agenda. Two sorts of entrepreneurs can use these tips:
Those who manage a team in a business and must ensure that everyone is working toward the same goals, upholding the same principles, and being inspired by the same future.
Those who are sole proprietors or independent contractors and who must maintain strict self-discipline in order to stay innovative in their industry and adhere to their own growth strategy.
Here's Steve Jobs's email outlining the annual meeting agenda. It's an 11-part summary of the company's shape and strategy.
Steve Jobs outlines Apple's 2011 strategy, 10/24/10
1. Correct your data
Business leaders must comprehend their company's metrics. Jobs either mentions critical information he already knows or demands slides showing the numbers he wants. These numbers fall under 2 categories:
Metrics for growth and strategy
As we will see, this was a crucial statistic for Apple since it signaled the beginning of the Post PC era and required them to make significant strategic changes in order to stay ahead of the curve. Post PC products now account for 66% of our revenues.
Within six months, iPad outsold Mac, another sign of the Post-PC age. As we will see, Jobs thought the iPad would be the next big thing, and item number four on the agenda is one of the most thorough references to the iPad.
Geographical analysis: Here, Jobs emphasizes China, where the corporation has a slower start than anticipated. China was dominating Apple's sales growth with 16% of revenue one year after this meeting.
Metrics for people & culture
The individuals that make up a firm are more significant to its success than its headcount or average age. That holds true regardless of size, from a 5-person startup to a Fortune 500 firm. Jobs was aware of this, which is why his suggested agenda begins by emphasizing demographic data.
Along with the senior advancements in the previous year's requested statistic, it's crucial to demonstrate that if the business is growing, the employees who make it successful must also grow.
2. Recognize the vulnerabilities and strengths of your rivals
Steve Jobs was known for attacking his competition in interviews and in his strategies and roadmaps. This agenda mentions 18 competitors, including:
Google 7 times
Android 3 times
Samsung 2 times
Jobs' agenda email was issued 6 days after Apple's Q4 results call (2010). On the call, Jobs trashed Google and Android. His 5-minute intervention included:
Google has acknowledged that the present iteration of Android is not tablet-optimized.
Future Android tablets will not work (Dead On Arrival)
While Google Play only has 90,000 apps, the Apple App Store has 300,000.
Android is extremely fragmented and is continuing to do so.
The App Store for iPad contains over 35,000 applications. The market share of the latest generation of tablets (which debuted in 2011) will be close to nil.
Jobs' aim in blasting the competition on that call was to reassure investors about the upcoming flood of new tablets. Jobs often criticized Google, Samsung, and Microsoft, but he also acknowledged when they did a better job. He was great at detecting his competitors' advantages and devising ways to catch up.
Jobs doesn't hold back when he says in bullet 1 of his agenda: "We further lock customers into our ecosystem while Google and Microsoft are further along on the technology, but haven't quite figured it out yet tie all of our goods together."
The plan outlined in bullet point 5 is immediately clear: catch up to Android where we are falling behind (notifications, tethering, and speech), and surpass them (Siri,). It's important to note that Siri frequently let users down and never quite lived up to expectations.
Regarding MobileMe, see Bullet 6 Jobs admits that when it comes to cloud services like contacts, calendars, and mail, Google is far ahead of Apple.
3. Adapt or perish
Steve Jobs was a visionary businessman. He knew personal computers were the future when he worked on the first Macintosh in the 1980s.
Jobs acknowledged the Post-PC age in his 2010 D8 interview.
Will the tablet replace the laptop, Walt Mossberg questioned Jobs? Jobs' response:
“You know, when we were an agrarian nation, all cars were trucks, because that’s what you needed on the farm. As vehicles started to be used in the urban centers and America started to move into those urban and suburban centers, cars got more popular and innovations like automatic transmission and things that you didn’t care about in a truck as much started to become paramount in cars. And now, maybe 1 out of every 25 vehicles is a truck, where it used to be 100%. PCs are going to be like trucks. They’re still going to be around, still going to have a lot of value, but they’re going to be used by one out of X people.”
Imagine how forward-thinking that was in 2010, especially for the Macintosh creator. You have to be willing to recognize that things were changing and that it was time to start over and focus on the next big thing.
Post-PC is priority number 8 in his 2010 agenda's 2011 Strategy section. Jobs says Apple is the first firm to get here and that Post PC items account about 66% of our income. The iPad outsold the Mac in 6 months, and the Post-PC age means increased mobility (smaller, thinner, lighter). Samsung had just introduced its first tablet, while Apple was working on the iPad 3. (as mentioned in bullet 4).
4. Plan ahead (and different)
Jobs' agenda warns that Apple risks clinging to outmoded paradigms. Clayton Christensen explains in The Innovators Dilemma that huge firms neglect disruptive technologies until they become profitable. Samsung's Galaxy tab, released too late, never caught up to Apple.
Apple faces a similar dilemma with the iPhone, its cash cow for over a decade. It doesn't sell as much because consumers aren't as excited about new iPhone launches and because technology is developing and cell phones may need to be upgraded.
Large companies' established consumer base typically hinders innovation. Clayton Christensen emphasizes that loyal customers from established brands anticipate better versions of current products rather than something altogether fresh and new technologies.
Apple's marketing is smart. Apple's ecosystem is trusted by customers, and its products integrate smoothly. So much so that Apple can afford to be a disruptor by doing something no one has ever done before, something the world's largest corporation shouldn't be the first to try. Apple can test the waters and produce a tremendous innovation tsunami, something few corporations can do.
In March 2011, Jobs appeared at an Apple event. During his address, Steve reminded us about Apple's brand:
“It’s in Apple’s DNA, that technology alone is not enough. That it’s technology married with liberal arts, married with the humanities that yields us the results that make our hearts sink. And nowhere is that more true that in these Post-PC devices.“
More than a decade later, Apple remains one of the most innovative and trailblazing companies in the Post-PC world (industry-disrupting products like Airpods or the Apple Watch came out after that 2011 strategy meeting), and it has reinvented how we use laptops with its M1-powered line of laptops offering unprecedented performance.
A decade after Jobs' death, Apple remains the world's largest firm, and its former CEO had a crucial part in its expansion. If you can do 1% of what Jobs did, you may be 1% as successful.
Not bad.
Josh Chesler
2 years ago
10 Sneaker Terms Every Beginner Should Know
So you want to get into sneakers? Buying a few sneakers and figuring it out seems simple. Then you miss out on the weekend's instant-sellout releases, so you head to eBay, Twitter, or your local sneaker group to see what's available, since you're probably not ready to pay Flight Club prices just yet.
That's when you're bombarded with new nicknames, abbreviations, and general sneaker slang. It would take months to explain every word and sneaker, so here's a starter kit of ten simple terms to get you started. (Yeah, mostly Jordan. Does anyone really start with Kith or Nike SB?)
10. Colorways
Colorways are a common term in fashion, design, and other visual fields. It's just the product's color scheme. In the case of sneakers, the colorway is often as important as the actual model. Are this year's "Chicago" Air Jordan 1s more durable than last year's "Black/Gum" colorway? Because of their colorway and rarity, the Chicagos are worth roughly three pairs of the Black/Gum kicks.
Pro Tip: A colorway with a well-known nickname is almost always worth more than one without, and the same goes for collaborations.
9. Beaters
A “beater” is a well-worn, likely older model of shoe that has significant wear and tear on it. Rarely sold with the original box or extra laces, beaters rarely sell for much. Unlike most “worn” sneakers, beaters are used for rainy days and the gym. It's exactly what it sounds like, a box full of beaters, and they're a good place to start if you're looking for some cheap old kicks.
Pro Tip: Know which shoes clean up nicely. The shape of lower top sneakers with wider profiles, like SB Dunk Lows and Air Jordan 3s, tends to hold better over time than their higher and narrower cousins.
8. Retro
In the world of Jordan Brand, a “Retro” release is simply a release (or re-release) of a colorway after the shoe model's initial release. For example, the original Air Jordan 7 was released in 1992, but the Bordeaux colorway was re-released in 2011 and recently (2015). An Air Jordan model is released every year, and while half of them are unpopular and unlikely to be Retroed soon, any of them could be re-released whenever Nike and Jordan felt like it.
Pro Tip: Now that the Air Jordan line has been around for so long, the model that tends to be heavily retroed in a year is whichever shoe came out 23 (Michael Jordan’s number during the prime of his career) years ago. The Air Jordan 6 (1991) got new colorways last year, the Air Jordan 7 this year, and more Air Jordan 8s will be released later this year and early next year (1993).
7. PP/Inv
In spite of the fact that eBay takes roughly 10% of the final price, many sneaker buyers and sellers prefer to work directly with PayPal. Selling sneakers for $100 via PayPal invoice or $100 via PayPal friends/family is common on social media. Because no one wants their eBay account suspended for promoting PayPal deals, many eBay sellers will simply state “Message me for a better price.”
Pro Tip: PayPal invoices protect buyers well, but gifting or using Google Wallet does not. Unless you're certain the seller is legitimate, only use invoiced goods/services payments.
6. Yeezy
Kanye West and his sneakers are known as Yeezys. The rapper's first two Yeezys were made by Nike before switching to Adidas. Everything Yeezy-related will be significantly more expensive (and therefore have significantly more fakes made). Not only is the Nike Air Yeezy 2 “Red October” one of the most sought-after sneakers, but the Yeezy influence can be seen everywhere.
Pro Tip: If you're going to buy Yeezys, make sure you buy them from a reputable retailer or reseller. With so many fakes out there, it's not worth spending a grand on something you're not 100% sure is real.
5. GR/Limited
Regardless of how visually repulsive, uncomfortable, and/or impractical a sneaker is, if it’s rare enough, people will still want it. GR stands for General Release, which means they're usually available at retail. Reselling a “Limited Edition” release is costly. Supply and demand, but in this case, the limited supply drives up demand. If you want to get some of the colorways made for rappers, NBA players (Player Exclusive or PE models), and other celebrities, be prepared to pay a premium.
Pro Tip: Limited edition sneakers, like the annual Doernbecher Freestyle sneakers Nike creates with kids from Portland's Doernbecher Children's Hospital, will always be more expensive and limited. Or, you can use automated sneaker-buying software.
4. Grails
A “grail” is a pair of sneakers that someone desires above all others. To obtain their personal grails, people are willing to pay significantly more than the retail price. There doesn't have to be any rhyme or reason why someone chose a specific pair as their grails.
Pro Tip: For those who don't have them, the OG "Bred" or "Royal" Air Jordan 1s, the "Concord" Air Jordan 11s, etc., are all grails.
3. Bred
Anything released in “Bred” (black and red) will sell out quickly. Most resale Air Jordans (and other sneakers) come in the Bred colorway, which is a fan favorite. Bred is a good choice for a first colorway, especially on a solid sneaker silhouette.
Pro Tip: Apart from satisfying the world's hypebeasts, Bred sneakers will probably match a lot of your closet.
2. DS
DS = Deadstock = New. That's it. If something has been worn or tried on, it is no longer DS. Very Near Deadstock (VNDS) Pass As Deadstock It's a cute way of saying your sneakers have been worn but are still in good shape. In the sneaker world, “worn” means they are no longer new, but not too old or beat up.
Pro Tip: Ask for photos of any marks or defects to see what you’re getting before you buy used shoes, also find out if they come with the original box and extra laces, because that can be a sign that they’re in better shape.
1. Fake/Unauthorized
The words “Unauthorized,” “Replica,” “B-grades,” and “Super Perfect” all mean the shoes are fake. It means they aren't made by the actual company, no matter how close or how good the quality. If that's what you want, go ahead and get them. Do not wear them if you do not want the rest of the sneaker world to mock them.
Pro Tip: If you’re not sure if shoes are real or not, do a “Legit Check” on Twitter or Facebook. You'll get dozens of responses in no time.

Josef Cruz
1 year ago
My friend worked in a startup scam that preys on slothful individuals.
He explained everything.
A drinking buddy confessed. Alexander. He says he works at a startup based on a scam, which appears too clever to be a lie.
Alexander (assuming he developed the story) or the startup's creator must have been a genius.
This is the story of an Internet scam that targets older individuals and generates tens of millions of dollars annually.
The business sells authentic things at 10% of their market value. This firm cannot be lucrative, but the entrepreneur has a plan: monthly subscriptions to a worthless service.
The firm can then charge the customer's credit card to settle the gap. The buyer must subscribe without knowing it. What's their strategy?
How does the con operate?
Imagine a website with a split homepage. On one page, the site offers an attractive goods at a ridiculous price (from 1 euro to 10% of the product's market worth).
Same product, but with a stupid monthly subscription. Business is unsustainable. They buy overpriced products and resell them too cheaply, hoping customers will subscribe to a useless service.
No customer will want this service. So they create another illegal homepage that hides the monthly subscription offer. After an endless scroll, a box says Yes, I want to subscribe to a service that costs x dollars per month.
Unchecking the checkbox bugs. When a customer buys a product on this page, he's enrolled in a monthly subscription. Not everyone should see it because it's illegal. So what does the startup do?
A page that varies based on the sort of website visitor, a possible consumer or someone who might be watching the startup's business
Startup technicians make sure the legal page is displayed when the site is accessed normally. Typing the web address in the browser, using Google, etc. The page crashes when buying a goods, preventing the purchase.
This avoids the startup from selling a product at a loss because the buyer won't subscribe to the worthless service and charge their credit card each month.
The illegal page only appears if a customer clicks on a Google ad, indicating interest in the offer.
Alexander says that a banker, police officer, or anyone else who visits the site (maybe for control) will only see a valid and buggy site as purchases won't be possible.
The latter will go to the site in the regular method (by typing the address in the browser, using Google, etc.) and not via an online ad.
Those who visit from ads are likely already lured by the site's price. They'll be sent to an illegal page that requires a subscription.
Laziness is humanity's secret weapon. The ordinary person ignores tiny monthly credit card charges. The subscription lasts around a year before the customer sees an unexpected deduction.
After-sales service (ASS) is useful in this situation.
After-sales assistance begins when a customer notices slight changes on his credit card, usually a year later.
The customer will search Google for the direct debit reference. How he'll complain to after-sales service.
It's crucial that ASS appears in the top 4/5 Google search results. This site must be clear, and offer chat, phone, etc., he argues.
The pigeon must be comforted after waking up. The customer learns via after-sales service that he subscribed to a service while buying the product, which justifies the debits on his card.
The customer will then clarify that he didn't intend to make the direct debits. The after-sales care professional will pretend to listen to the customer's arguments and complaints, then offer to unsubscribe him for free because his predicament has affected him.
In 99% of cases, the consumer is satisfied since the after-sales support unsubscribed him for free, and he forgets the debited amounts.
The remaining 1% is split between 0.99% who are delighted to be reimbursed and 0.01%. We'll pay until they're done. The customer should be delighted, not object or complain, and keep us beneath the radar (their situation is resolved, the rest, they don’t care).
It works, so we expand our thinking.
Startup has considered industrialization. Since this fraud is working, try another. Automate! So they used a site generator (only for product modifications), underpaid phone operators for after-sales service, and interns for fresh product ideas.
The company employed a data scientist. This has allowed the startup to recognize that specific customer profiles can be re-registered in the database and that it will take X months before they realize they're subscribing to a worthless service. Customers are re-subscribed to another service, then unsubscribed before realizing it.
Alexander took months to realize the deception and leave. Lawyers and others apparently threatened him and former colleagues who tried to talk about it.
The startup would have earned prizes and competed in contests. He adds they can provide evidence to any consumer group, media, police/gendarmerie, or relevant body. When I submitted my information to the FBI, I was told, "We know, we can't do much.", he says.